Notice
Recent Posts
Recent Comments
Link
일 | 월 | 화 | 수 | 목 | 금 | 토 |
---|---|---|---|---|---|---|
1 | 2 | 3 | 4 | 5 | ||
6 | 7 | 8 | 9 | 10 | 11 | 12 |
13 | 14 | 15 | 16 | 17 | 18 | 19 |
20 | 21 | 22 | 23 | 24 | 25 | 26 |
27 | 28 | 29 | 30 |
Tags
- dataframe
- 제어문
- 넘파이
- KMeans Clustering
- 파이썬 크롤링
- 사이킷런
- NumPy
- 판다스
- Naive Bayes
- 파이썬 객체 지향 프로그래밍
- pandas
- 배열
- control statement
- 순회 크롤러
- python control statement
- 나이브베이즈
- sklearn
- 파이썬
- scikit-learn
- 타이타닉 데이터
- Machine Learning
- Data pre-processing
- 머신러닝
- 파이썬 제어문
- Python crawler
- K평균군집화
- python
- 파이썬 크롤러
- ML
- Titanic data set
Archives
- Today
- Total
Try to 개발자 EthanJ의 성장 로그
scikit-learn Machine Learning Logistic Regression 사이킷런 머신러닝 로지스틱 회귀 본문
CS & DS/scikit-learn Machine Learning
scikit-learn Machine Learning Logistic Regression 사이킷런 머신러닝 로지스틱 회귀
EthanJ 2022. 11. 15. 09:01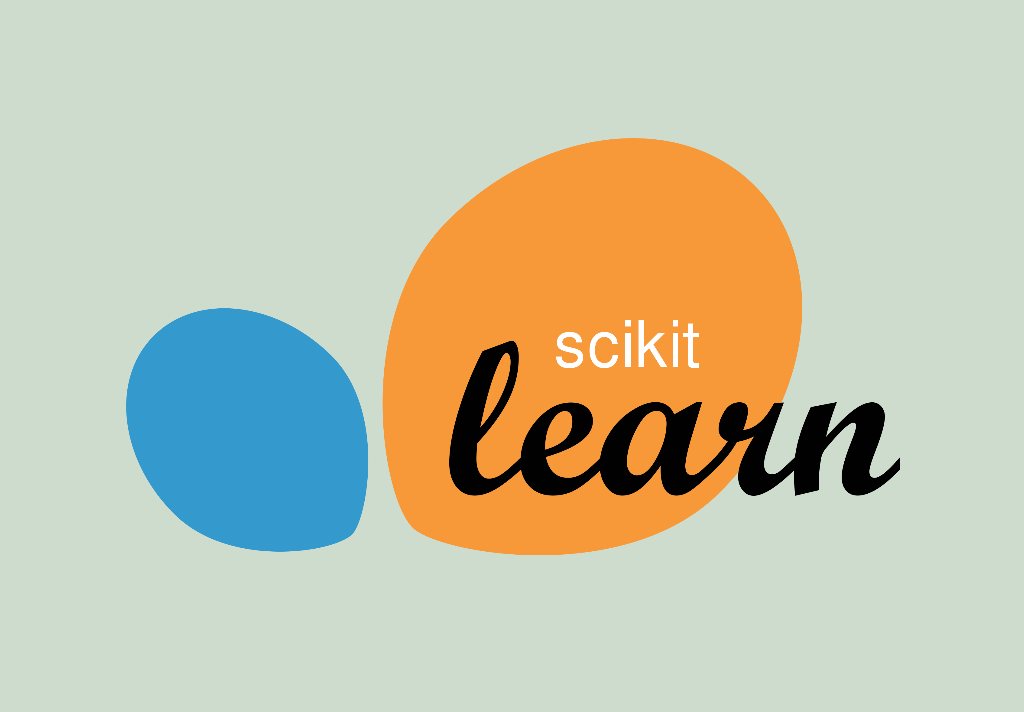
scikit-learn Machine Learning Logistic Regression: Predict Titanic Survived
사이킷런 머신러닝 로지스틱 회귀: 타이타닉 생존자 예측
In [1]:
import pandas as pd
# DataFrame float값을 소수점 두 번째 자리 까지만 표시
pd.options.display.float_format = '{:.2f}'.format
1. Data Collection¶
In [2]:
# Kaggle
# https://www.kaggle.com/competitions/titanic
file_url = "https://raw.githubusercontent.com/dev-EthanJ/scikit-learn_Machine_Learning/main/data/"
# 맨 첫 번째 줄 PassengerId를 Index 열로 지정
train = pd.read_csv(f'{file_url}/titanic_train.csv', index_col=0)
submission = pd.read_csv(f'{file_url}/titanic_test.csv', index_col=0)
In [3]:
train.head()
Out[3]:
Survived | Pclass | Name | Sex | Age | SibSp | Parch | Ticket | Fare | Cabin | Embarked | |
---|---|---|---|---|---|---|---|---|---|---|---|
PassengerId | |||||||||||
1 | 0 | 3 | Braund, Mr. Owen Harris | male | 22.00 | 1 | 0 | A/5 21171 | 7.25 | NaN | S |
2 | 1 | 1 | Cumings, Mrs. John Bradley (Florence Briggs Th... | female | 38.00 | 1 | 0 | PC 17599 | 71.28 | C85 | C |
3 | 1 | 3 | Heikkinen, Miss. Laina | female | 26.00 | 0 | 0 | STON/O2. 3101282 | 7.92 | NaN | S |
4 | 1 | 1 | Futrelle, Mrs. Jacques Heath (Lily May Peel) | female | 35.00 | 1 | 0 | 113803 | 53.10 | C123 | S |
5 | 0 | 3 | Allen, Mr. William Henry | male | 35.00 | 0 | 0 | 373450 | 8.05 | NaN | S |
In [4]:
submission.head()
Out[4]:
Pclass | Name | Sex | Age | SibSp | Parch | Ticket | Fare | Cabin | Embarked | |
---|---|---|---|---|---|---|---|---|---|---|
PassengerId | ||||||||||
892 | 3 | Kelly, Mr. James | male | 34.50 | 0 | 0 | 330911 | 7.83 | NaN | Q |
893 | 3 | Wilkes, Mrs. James (Ellen Needs) | female | 47.00 | 1 | 0 | 363272 | 7.00 | NaN | S |
894 | 2 | Myles, Mr. Thomas Francis | male | 62.00 | 0 | 0 | 240276 | 9.69 | NaN | Q |
895 | 3 | Wirz, Mr. Albert | male | 27.00 | 0 | 0 | 315154 | 8.66 | NaN | S |
896 | 3 | Hirvonen, Mrs. Alexander (Helga E Lindqvist) | female | 22.00 | 1 | 1 | 3101298 | 12.29 | NaN | S |
In [5]:
train.info()
<class 'pandas.core.frame.DataFrame'> Int64Index: 891 entries, 1 to 891 Data columns (total 11 columns): # Column Non-Null Count Dtype --- ------ -------------- ----- 0 Survived 891 non-null int64 1 Pclass 891 non-null int64 2 Name 891 non-null object 3 Sex 891 non-null object 4 Age 714 non-null float64 5 SibSp 891 non-null int64 6 Parch 891 non-null int64 7 Ticket 891 non-null object 8 Fare 891 non-null float64 9 Cabin 204 non-null object 10 Embarked 889 non-null object dtypes: float64(2), int64(4), object(5) memory usage: 83.5+ KB
독립변수
- Pclass : 티켓 클래스
- SibSp : 동승한 형제(Siblings)와 배우자(Spouse) 수
- Parch : 동승한 부모(Parents)와 자식들(Children) 수
- Ticket : 티켓 번호
- Fare : 요금
- Cabin : 호실
- Embarked : 승선한 항구
종속변수
- Survived : 생존 여부
In [6]:
# 기술통계
train.describe()
Out[6]:
Survived | Pclass | Age | SibSp | Parch | Fare | |
---|---|---|---|---|---|---|
count | 891.00 | 891.00 | 714.00 | 891.00 | 891.00 | 891.00 |
mean | 0.38 | 2.31 | 29.70 | 0.52 | 0.38 | 32.20 |
std | 0.49 | 0.84 | 14.53 | 1.10 | 0.81 | 49.69 |
min | 0.00 | 1.00 | 0.42 | 0.00 | 0.00 | 0.00 |
25% | 0.00 | 2.00 | 20.12 | 0.00 | 0.00 | 7.91 |
50% | 0.00 | 3.00 | 28.00 | 0.00 | 0.00 | 14.45 |
75% | 1.00 | 3.00 | 38.00 | 1.00 | 0.00 | 31.00 |
max | 1.00 | 3.00 | 80.00 | 8.00 | 6.00 | 512.33 |
Dtype=object
통계 정보:df.describe(include=["O"])
In [7]:
train.describe(include=["O"])
Out[7]:
Name | Sex | Ticket | Cabin | Embarked | |
---|---|---|---|---|---|
count | 891 | 891 | 891 | 204 | 889 |
unique | 891 | 2 | 681 | 147 | 3 |
top | Braund, Mr. Owen Harris | male | 347082 | B96 B98 | S |
freq | 1 | 577 | 7 | 4 | 644 |
1.1. 상관관계: df.corr()
¶
- 상관관계 강도 해석 (절댓값)
- 0.2 이하 : 상관관계가 거의 없음
- 0.2 ~ 0.4 : 낮은 상관관계
- 0.4 ~ 0.6 : 중간 상관관계
- 0.6 ~ 0.8 : 높은 상관관계
- 0.8 이상 : 매우 높은 상관관계
In [8]:
train.corr()
Out[8]:
Survived | Pclass | Age | SibSp | Parch | Fare | |
---|---|---|---|---|---|---|
Survived | 1.00 | -0.34 | -0.08 | -0.04 | 0.08 | 0.26 |
Pclass | -0.34 | 1.00 | -0.37 | 0.08 | 0.02 | -0.55 |
Age | -0.08 | -0.37 | 1.00 | -0.31 | -0.19 | 0.10 |
SibSp | -0.04 | 0.08 | -0.31 | 1.00 | 0.41 | 0.16 |
Parch | 0.08 | 0.02 | -0.19 | 0.41 | 1.00 | 0.22 |
Fare | 0.26 | -0.55 | 0.10 | 0.16 | 0.22 | 1.00 |
In [9]:
import matplotlib.pyplot as plt
import seaborn as sns
In [10]:
sns.heatmap(train.corr())
plt.show()
In [11]:
sns.heatmap(train.corr(), cmap='coolwarm')
plt.show()
In [12]:
sns.heatmap(train.corr(), cmap='coolwarm', vmin=-1, vmax=1)
plt.show()
In [13]:
sns.heatmap(train.corr(), cmap='coolwarm', vmin=-1, vmax=1, annot=True)
plt.show()
2. Data pre-processing¶
2.1. 결측치 처리¶
In [14]:
# Int64Index: 891 entries, Embarked: 889개 > 결측치 존재
train.info()
<class 'pandas.core.frame.DataFrame'> Int64Index: 891 entries, 1 to 891 Data columns (total 11 columns): # Column Non-Null Count Dtype --- ------ -------------- ----- 0 Survived 891 non-null int64 1 Pclass 891 non-null int64 2 Name 891 non-null object 3 Sex 891 non-null object 4 Age 714 non-null float64 5 SibSp 891 non-null int64 6 Parch 891 non-null int64 7 Ticket 891 non-null object 8 Fare 891 non-null float64 9 Cabin 204 non-null object 10 Embarked 889 non-null object dtypes: float64(2), int64(4), object(5) memory usage: 83.5+ KB
In [15]:
# 결측치 갯수 확인
train.isnull().sum()
Out[15]:
Survived 0 Pclass 0 Name 0 Sex 0 Age 177 SibSp 0 Parch 0 Ticket 0 Fare 0 Cabin 687 Embarked 2 dtype: int64
In [16]:
train.Embarked.isna().sum()
Out[16]:
2
Embarked
: 최빈값 대체¶
- 최빈값 'S'로 결측치 채움
In [17]:
train.Embarked = train.Embarked.fillna("S")
train.Embarked.unique()
Out[17]:
array(['S', 'C', 'Q'], dtype=object)
Age
: 평균 연령 대체¶
- 이름에서 경칭(title) 추출 → title별 평균 연령으로 적용
In [18]:
train['Title'] = train.Name.str.extract('([A-Za-z]+)\.')
train.Title.head()
Out[18]:
PassengerId 1 Mr 2 Mrs 3 Miss 4 Mrs 5 Mr Name: Title, dtype: object
In [19]:
train['Title'].value_counts()
Out[19]:
Mr 517 Miss 182 Mrs 125 Master 40 Dr 7 Rev 6 Mlle 2 Major 2 Col 2 Countess 1 Capt 1 Ms 1 Sir 1 Lady 1 Mme 1 Don 1 Jonkheer 1 Name: Title, dtype: int64
In [20]:
train['Title'].unique()
Out[20]:
array(['Mr', 'Mrs', 'Miss', 'Master', 'Don', 'Rev', 'Dr', 'Mme', 'Ms', 'Major', 'Lady', 'Sir', 'Mlle', 'Col', 'Capt', 'Countess', 'Jonkheer'], dtype=object)
- 10개 미만의
Title
걸러내기
In [21]:
rare_list = list()
for title in set(train['Title']):
if list(train['Title']).count(title) < 10:
rare_list.append(title)
rare_list
Out[21]:
['Don', 'Countess', 'Mme', 'Capt', 'Mlle', 'Dr', 'Major', 'Rev', 'Lady', 'Sir', 'Jonkheer', 'Col', 'Ms']
Rare
로 값 변경
In [22]:
train['Title'] = train['Title'].replace(rare_list, 'Rare')
train.Title.value_counts()
Out[22]:
Mr 517 Miss 182 Mrs 125 Master 40 Rare 27 Name: Title, dtype: int64
In [23]:
title_age_mean = train.groupby('Title')['Age'].mean()
title_age_mean
Out[23]:
Title Master 4.57 Miss 21.77 Mr 32.37 Mrs 35.90 Rare 42.38 Name: Age, dtype: float64
- 각
Title
별Age
평균값으로 결측치 채우기
In [24]:
for record in train['Title'].unique():
train.loc[(train.Age.isnull()) & (train.Title == record), 'Age'] = title_age_mean[record]
In [25]:
train.isnull().sum()
Out[25]:
Survived 0 Pclass 0 Name 0 Sex 0 Age 0 SibSp 0 Parch 0 Ticket 0 Fare 0 Cabin 687 Embarked 0 Title 0 dtype: int64
df.drop([columns list])
¶
- 유의미한 차이(종속변수에 영향)를 보기 힘든 column drop
In [26]:
train[['Name', 'Ticket', 'Cabin']].head()
Out[26]:
Name | Ticket | Cabin | |
---|---|---|---|
PassengerId | |||
1 | Braund, Mr. Owen Harris | A/5 21171 | NaN |
2 | Cumings, Mrs. John Bradley (Florence Briggs Th... | PC 17599 | C85 |
3 | Heikkinen, Miss. Laina | STON/O2. 3101282 | NaN |
4 | Futrelle, Mrs. Jacques Heath (Lily May Peel) | 113803 | C123 |
5 | Allen, Mr. William Henry | 373450 | NaN |
In [27]:
train = train.drop(columns=['Name', 'Ticket', 'Cabin'])
train.head()
Out[27]:
Survived | Pclass | Sex | Age | SibSp | Parch | Fare | Embarked | Title | |
---|---|---|---|---|---|---|---|---|---|
PassengerId | |||||||||
1 | 0 | 3 | male | 22.00 | 1 | 0 | 7.25 | S | Mr |
2 | 1 | 1 | female | 38.00 | 1 | 0 | 71.28 | C | Mrs |
3 | 1 | 3 | female | 26.00 | 0 | 0 | 7.92 | S | Miss |
4 | 1 | 1 | female | 35.00 | 1 | 0 | 53.10 | S | Mrs |
5 | 0 | 3 | male | 35.00 | 0 | 0 | 8.05 | S | Mr |
In [28]:
train.info()
<class 'pandas.core.frame.DataFrame'> Int64Index: 891 entries, 1 to 891 Data columns (total 9 columns): # Column Non-Null Count Dtype --- ------ -------------- ----- 0 Survived 891 non-null int64 1 Pclass 891 non-null int64 2 Sex 891 non-null object 3 Age 891 non-null float64 4 SibSp 891 non-null int64 5 Parch 891 non-null int64 6 Fare 891 non-null float64 7 Embarked 891 non-null object 8 Title 891 non-null object dtypes: float64(2), int64(4), object(3) memory usage: 69.6+ KB
2.2. 범주형 변수(categorical data) 변환¶
In [29]:
train_df = pd.get_dummies(train,
columns=['Sex', 'Title', 'Embarked'], drop_first=True)
train_df.head()
Out[29]:
Survived | Pclass | Age | SibSp | Parch | Fare | Sex_male | Title_Miss | Title_Mr | Title_Mrs | Title_Rare | Embarked_Q | Embarked_S | |
---|---|---|---|---|---|---|---|---|---|---|---|---|---|
PassengerId | |||||||||||||
1 | 0 | 3 | 22.00 | 1 | 0 | 7.25 | 1 | 0 | 1 | 0 | 0 | 0 | 1 |
2 | 1 | 1 | 38.00 | 1 | 0 | 71.28 | 0 | 0 | 0 | 1 | 0 | 0 | 0 |
3 | 1 | 3 | 26.00 | 0 | 0 | 7.92 | 0 | 1 | 0 | 0 | 0 | 0 | 1 |
4 | 1 | 1 | 35.00 | 1 | 0 | 53.10 | 0 | 0 | 0 | 1 | 0 | 0 | 1 |
5 | 0 | 3 | 35.00 | 0 | 0 | 8.05 | 1 | 0 | 1 | 0 | 0 | 0 | 1 |
- 하나의 함수
pre_processing
으로 정리
In [30]:
def pre_processing(df : pd.DataFrame):
df.Embarked = df.Embarked.fillna("S")
df.Fare = df.Fare.fillna(0)
df['Title'] = df.Name.str.extract('([A-Za-z]+)\.')
rarelist = [a for a in set(df['Title'])
if list(df['Title']).count(a) < 10]
df['Title'] = df['Title'].replace(rarelist, 'Rare')
title_age_mean = df.groupby(['Title'])['Age'].mean()
for v in df['Title'].unique():
df.loc[df.Age.isnull() & (df.Title == v), 'Age'] = title_age_mean[v]
df_clean = df.drop(columns=['Name', 'Ticket', 'Cabin'])
return pd.get_dummies(df_clean,
columns = ['Sex', 'Embarked','Title'], drop_first=True)
3. Training Data¶
In [31]:
from sklearn.linear_model import LogisticRegression
model = LogisticRegression()
In [32]:
from sklearn.model_selection import train_test_split
X = train_df.drop(columns=['Survived'])
y = train_df.Survived
X_train, X_test, y_train, y_test = train_test_split(
X, y, test_size = 0.2, random_state = 100
)
3.1. Fitting Model (모델 학습)¶
In [33]:
model.fit(X_train, y_train)
/usr/local/lib/python3.7/dist-packages/sklearn/linear_model/_logistic.py:818: ConvergenceWarning: lbfgs failed to converge (status=1): STOP: TOTAL NO. of ITERATIONS REACHED LIMIT. Increase the number of iterations (max_iter) or scale the data as shown in: https://scikit-learn.org/stable/modules/preprocessing.html Please also refer to the documentation for alternative solver options: https://scikit-learn.org/stable/modules/linear_model.html#logistic-regression extra_warning_msg=_LOGISTIC_SOLVER_CONVERGENCE_MSG,
Out[33]:
LogisticRegression()
3.2. Model Predict (모델 예측)¶
In [34]:
pred = model.predict(X_test)
- 모델 평가: 로지스틱 회귀 > 분류 문제
4. Evaluating Model¶
accracy_score(y_test, pred)
: 예측값과 실제값을 비교하여 얼마나 맞추었는지 확인
In [35]:
from sklearn.metrics import accuracy_score
accuracy_score(y_test, pred)
Out[35]:
0.8268156424581006
In [36]:
model.coef_
Out[36]:
array([[-1.10188806, -0.0400297 , -0.35743286, -0.3701925 , 0.00382998, -0.11414246, 0.80872633, -1.86843605, 1.40438893, -1.14529314, -0.14389453, -0.33569188]])
In [37]:
pd.options.display.float_format = '{:.4f}'.format
# model.coef_: 이중 list: indexing [0] 필요
pd.Series(model.coef_[0], index = X.columns)
Out[37]:
Pclass -1.1019 Age -0.0400 SibSp -0.3574 Parch -0.3702 Fare 0.0038 Sex_male -0.1141 Title_Miss 0.8087 Title_Mr -1.8684 Title_Mrs 1.4044 Title_Rare -1.1453 Embarked_Q -0.1439 Embarked_S -0.3357 dtype: float64
5. Addtional Information¶
피처 엔지니어링 (Feature Engineering)¶
기존 데이터를 손보아 더 나은 변수를 만드는 기법
- e.g. 더미 변수
도메인 지식의 활용이 중요
데이터에 사전 지식이 있으면 어떤 변수를 어떻게 바꾸면 더 나은 피처를 얻을 수 있을지 파악 가능
도메인 지식을 바탕으로 정확한 목적을 가지고 수행하는 피처 엔지니어링이 더욱 효율적
다중공선성(Multicolinearity) 문제¶
선형 회귀 분석, 로지스틱 회귀 분석 등 선형 모델에서 독립변수 사이에 상관관계가 높은 때에 발생하는 문제
특정 독립변수 간의 상관관계가 매우 높다면 종속변수의 변화 요인이 정확히 어떤 변수 때문인지 불투명해짐
선형 모델은 독립변수 간의 독립성을 전제로 하기 때문에, 다중공선성 문제를 해결해주는 것이 좋음
Solution
상관관계가 높은 변수 중 하나를 제거
둘을 모두 포괄시키는 새로운 변수 생성
PCA 등의 방법으로 차원 축소를 수행
- PCA(Principal Component Analysis, 주성분 분석)
6. Logistic Regression: 로지스틱 회귀¶
- 로지스틱 함수 Logistic function 를 사용하여 선형 회귀 분석의 직선 형태를 1과 0에 한없이 가까워지는 곡선 형태로 바꿔줌
- 기본적으로 0.5를 기준으로 1과 0으로 변환시킨 값을 보여줌
7. Kaggle Competition submission: Deployment Model¶
In [38]:
submission.head()
Out[38]:
Pclass | Name | Sex | Age | SibSp | Parch | Ticket | Fare | Cabin | Embarked | |
---|---|---|---|---|---|---|---|---|---|---|
PassengerId | ||||||||||
892 | 3 | Kelly, Mr. James | male | 34.5000 | 0 | 0 | 330911 | 7.8292 | NaN | Q |
893 | 3 | Wilkes, Mrs. James (Ellen Needs) | female | 47.0000 | 1 | 0 | 363272 | 7.0000 | NaN | S |
894 | 2 | Myles, Mr. Thomas Francis | male | 62.0000 | 0 | 0 | 240276 | 9.6875 | NaN | Q |
895 | 3 | Wirz, Mr. Albert | male | 27.0000 | 0 | 0 | 315154 | 8.6625 | NaN | S |
896 | 3 | Hirvonen, Mrs. Alexander (Helga E Lindqvist) | female | 22.0000 | 1 | 1 | 3101298 | 12.2875 | NaN | S |
In [39]:
sub_df = pre_processing(submission)
sub_df.head()
Out[39]:
Pclass | Age | SibSp | Parch | Fare | Sex_male | Embarked_Q | Embarked_S | Title_Miss | Title_Mr | Title_Mrs | Title_Rare | |
---|---|---|---|---|---|---|---|---|---|---|---|---|
PassengerId | ||||||||||||
892 | 3 | 34.5000 | 0 | 0 | 7.8292 | 1 | 1 | 0 | 0 | 1 | 0 | 0 |
893 | 3 | 47.0000 | 1 | 0 | 7.0000 | 0 | 0 | 1 | 0 | 0 | 1 | 0 |
894 | 2 | 62.0000 | 0 | 0 | 9.6875 | 1 | 1 | 0 | 0 | 1 | 0 | 0 |
895 | 3 | 27.0000 | 0 | 0 | 8.6625 | 1 | 0 | 1 | 0 | 1 | 0 | 0 |
896 | 3 | 22.0000 | 1 | 1 | 12.2875 | 0 | 0 | 1 | 0 | 0 | 1 | 0 |
In [40]:
sub_pred = model.predict(sub_df)
sub_pred
/usr/local/lib/python3.7/dist-packages/sklearn/base.py:493: FutureWarning: The feature names should match those that were passed during fit. Starting version 1.2, an error will be raised. Feature names must be in the same order as they were in fit. warnings.warn(message, FutureWarning)
Out[40]:
array([0, 0, 0, 0, 0, 0, 1, 0, 1, 0, 0, 0, 1, 0, 0, 1, 1, 0, 0, 0, 0, 0, 0, 1, 1, 0, 1, 0, 0, 0, 0, 0, 0, 0, 1, 0, 0, 0, 0, 0, 0, 0, 0, 0, 0, 0, 1, 0, 1, 0, 0, 0, 0, 1, 0, 0, 0, 0, 0, 1, 0, 0, 0, 1, 1, 0, 1, 0, 1, 0, 1, 0, 0, 1, 1, 1, 0, 0, 0, 1, 0, 0, 0, 0, 1, 0, 1, 0, 0, 0, 0, 0, 0, 0, 1, 0, 0, 0, 0, 0, 1, 0, 0, 0, 0, 0, 0, 0, 0, 0, 0, 1, 1, 1, 0, 0, 0, 0, 1, 0, 1, 0, 1, 0, 0, 0, 0, 1, 0, 0, 0, 1, 0, 0, 0, 0, 0, 0, 0, 0, 0, 1, 0, 0, 0, 0, 0, 0, 0, 0, 1, 0, 0, 0, 0, 0, 1, 0, 0, 0, 1, 0, 1, 0, 0, 0, 1, 0, 1, 0, 0, 0, 0, 0, 0, 1, 1, 0, 0, 1, 0, 1, 1, 0, 1, 0, 1, 0, 0, 0, 0, 0, 0, 0, 0, 0, 1, 0, 0, 0, 1, 0, 1, 1, 0, 1, 1, 0, 1, 0, 0, 0, 0, 0, 0, 0, 1, 0, 1, 0, 1, 0, 0, 0, 1, 0, 0, 1, 0, 0, 0, 1, 0, 0, 1, 0, 0, 0, 1, 1, 1, 0, 0, 0, 0, 0, 0, 0, 0, 0, 1, 0, 1, 0, 0, 0, 0, 0, 1, 0, 0, 0, 0, 0, 0, 0, 0, 0, 0, 0, 1, 0, 1, 1, 0, 1, 0, 0, 0, 0, 0, 0, 1, 1, 0, 0, 0, 0, 0, 0, 0, 1, 0, 0, 0, 0, 1, 0, 0, 0, 0, 0, 0, 0, 1, 0, 0, 0, 0, 0, 0, 0, 0, 1, 1, 1, 0, 0, 0, 0, 0, 0, 0, 0, 1, 0, 1, 1, 0, 0, 0, 1, 0, 0, 0, 0, 0, 0, 0, 1, 0, 0, 0, 1, 0, 0, 0, 0, 0, 1, 1, 0, 0, 0, 0, 0, 0, 0, 0, 0, 0, 1, 0, 0, 1, 0, 0, 0, 1, 0, 0, 1, 0, 0, 0, 1, 0, 0, 0, 0, 0, 0, 0, 0, 0, 0, 0, 0, 0, 0, 0, 0, 0, 0, 0, 1, 0, 1, 0, 0, 1, 0, 1, 0, 0, 1, 0, 1, 1, 0, 1, 1, 0, 0, 1, 0, 0, 0])
In [41]:
result = pd.DataFrame({'PassengerId':sub_df.index,'Survived':sub_pred})
result
Out[41]:
PassengerId | Survived | |
---|---|---|
0 | 892 | 0 |
1 | 893 | 0 |
2 | 894 | 0 |
3 | 895 | 0 |
4 | 896 | 0 |
... | ... | ... |
413 | 1305 | 0 |
414 | 1306 | 1 |
415 | 1307 | 0 |
416 | 1308 | 0 |
417 | 1309 | 0 |
418 rows × 2 columns
In [42]:
result.to_csv('titanic_submission.csv', index=False)
In [43]:
!pip install mlxtend
Looking in indexes: https://pypi.org/simple, https://us-python.pkg.dev/colab-wheels/public/simple/ Requirement already satisfied: mlxtend in /usr/local/lib/python3.7/dist-packages (0.14.0) Requirement already satisfied: scipy>=0.17 in /usr/local/lib/python3.7/dist-packages (from mlxtend) (1.7.3) Requirement already satisfied: numpy>=1.10.4 in /usr/local/lib/python3.7/dist-packages (from mlxtend) (1.21.6) Requirement already satisfied: matplotlib>=1.5.1 in /usr/local/lib/python3.7/dist-packages (from mlxtend) (3.2.2) Requirement already satisfied: scikit-learn>=0.18 in /usr/local/lib/python3.7/dist-packages (from mlxtend) (1.0.2) Requirement already satisfied: setuptools in /usr/local/lib/python3.7/dist-packages (from mlxtend) (57.4.0) Requirement already satisfied: pandas>=0.17.1 in /usr/local/lib/python3.7/dist-packages (from mlxtend) (1.3.5) Requirement already satisfied: cycler>=0.10 in /usr/local/lib/python3.7/dist-packages (from matplotlib>=1.5.1->mlxtend) (0.11.0) Requirement already satisfied: python-dateutil>=2.1 in /usr/local/lib/python3.7/dist-packages (from matplotlib>=1.5.1->mlxtend) (2.8.2) Requirement already satisfied: pyparsing!=2.0.4,!=2.1.2,!=2.1.6,>=2.0.1 in /usr/local/lib/python3.7/dist-packages (from matplotlib>=1.5.1->mlxtend) (3.0.9) Requirement already satisfied: kiwisolver>=1.0.1 in /usr/local/lib/python3.7/dist-packages (from matplotlib>=1.5.1->mlxtend) (1.4.4) Requirement already satisfied: typing-extensions in /usr/local/lib/python3.7/dist-packages (from kiwisolver>=1.0.1->matplotlib>=1.5.1->mlxtend) (4.1.1) Requirement already satisfied: pytz>=2017.3 in /usr/local/lib/python3.7/dist-packages (from pandas>=0.17.1->mlxtend) (2022.6) Requirement already satisfied: six>=1.5 in /usr/local/lib/python3.7/dist-packages (from python-dateutil>=2.1->matplotlib>=1.5.1->mlxtend) (1.15.0) Requirement already satisfied: threadpoolctl>=2.0.0 in /usr/local/lib/python3.7/dist-packages (from scikit-learn>=0.18->mlxtend) (3.1.0) Requirement already satisfied: joblib>=0.11 in /usr/local/lib/python3.7/dist-packages (from scikit-learn>=0.18->mlxtend) (1.2.0)
In [44]:
import joblib
joblib.dump(model, 'logistic_regression_titanic.pkl')
Out[44]:
['logistic_regression_titanic.pkl']
In [45]:
model_from_joblib = joblib.load('logistic_regression_titanic.pkl')
pd.Series(model_from_joblib.coef_[0], index=X.columns)
Out[45]:
Pclass -1.1019 Age -0.0400 SibSp -0.3574 Parch -0.3702 Fare 0.0038 Sex_male -0.1141 Title_Miss 0.8087 Title_Mr -1.8684 Title_Mrs 1.4044 Title_Rare -1.1453 Embarked_Q -0.1439 Embarked_S -0.3357 dtype: float64
8. Manipulating Data & Retraining¶
8.1. SibSp
& Parch
→ Family
변수화¶
In [46]:
train_df['Family'] = train_df['SibSp'] + train_df['Parch'] + 1
train_df.Family.head()
Out[46]:
PassengerId 1 2 2 2 3 1 4 2 5 1 Name: Family, dtype: int64
In [47]:
train_df = train_df.drop(['SibSp', 'Parch'], axis=1)
train_df.head()
Out[47]:
Survived | Pclass | Age | Fare | Sex_male | Title_Miss | Title_Mr | Title_Mrs | Title_Rare | Embarked_Q | Embarked_S | Family | |
---|---|---|---|---|---|---|---|---|---|---|---|---|
PassengerId | ||||||||||||
1 | 0 | 3 | 22.0000 | 7.2500 | 1 | 0 | 1 | 0 | 0 | 0 | 1 | 2 |
2 | 1 | 1 | 38.0000 | 71.2833 | 0 | 0 | 0 | 1 | 0 | 0 | 0 | 2 |
3 | 1 | 3 | 26.0000 | 7.9250 | 0 | 1 | 0 | 0 | 0 | 0 | 1 | 1 |
4 | 1 | 1 | 35.0000 | 53.1000 | 0 | 0 | 0 | 1 | 0 | 0 | 1 | 2 |
5 | 0 | 3 | 35.0000 | 8.0500 | 1 | 0 | 1 | 0 | 0 | 0 | 1 | 1 |
In [48]:
X = train_df.drop(columns=['Survived'])
y = train_df.Survived
X_train, X_test, y_train, y_test = train_test_split(
X, y, test_size=0.2, random_state=100
)
In [49]:
new_model = LogisticRegression()
new_model.fit(X_train, y_train)
/usr/local/lib/python3.7/dist-packages/sklearn/linear_model/_logistic.py:818: ConvergenceWarning: lbfgs failed to converge (status=1): STOP: TOTAL NO. of ITERATIONS REACHED LIMIT. Increase the number of iterations (max_iter) or scale the data as shown in: https://scikit-learn.org/stable/modules/preprocessing.html Please also refer to the documentation for alternative solver options: https://scikit-learn.org/stable/modules/linear_model.html#logistic-regression extra_warning_msg=_LOGISTIC_SOLVER_CONVERGENCE_MSG,
Out[49]:
LogisticRegression()
In [50]:
pred = new_model.predict(X_test)
accuracy_score(y_test, pred)
Out[50]:
0.8268156424581006
In [51]:
pd.Series(new_model.coef_[0], index=X.columns)
Out[51]:
Pclass -1.0664 Age -0.0374 Fare 0.0041 Sex_male -0.1957 Title_Miss 0.7346 Title_Mr -1.8435 Title_Mrs 1.4822 Title_Rare -1.1388 Embarked_Q -0.2150 Embarked_S -0.2693 Family -0.3741 dtype: float64
'CS & DS > scikit-learn Machine Learning' 카테고리의 다른 글
Comments