일 | 월 | 화 | 수 | 목 | 금 | 토 |
---|---|---|---|---|---|---|
1 | 2 | 3 | 4 | 5 | ||
6 | 7 | 8 | 9 | 10 | 11 | 12 |
13 | 14 | 15 | 16 | 17 | 18 | 19 |
20 | 21 | 22 | 23 | 24 | 25 | 26 |
27 | 28 | 29 | 30 |
- 나이브베이즈
- sklearn
- 타이타닉 데이터
- Machine Learning
- dataframe
- Naive Bayes
- pandas
- NumPy
- 사이킷런
- 파이썬 크롤러
- 머신러닝
- 배열
- 파이썬 제어문
- 순회 크롤러
- 제어문
- control statement
- K평균군집화
- Titanic data set
- Python crawler
- KMeans Clustering
- 파이썬 크롤링
- 파이썬
- python control statement
- 파이썬 객체 지향 프로그래밍
- 넘파이
- 판다스
- scikit-learn
- python
- ML
- Data pre-processing
- Today
- Total
Try to 개발자 EthanJ의 성장 로그
scikit-learn Machine Learning XGBoost 사이킷런 머신러닝 XGBoost 본문
scikit-learn Machine Learning XGBoost 사이킷런 머신러닝 XGBoost
EthanJ 2022. 11. 21. 14:50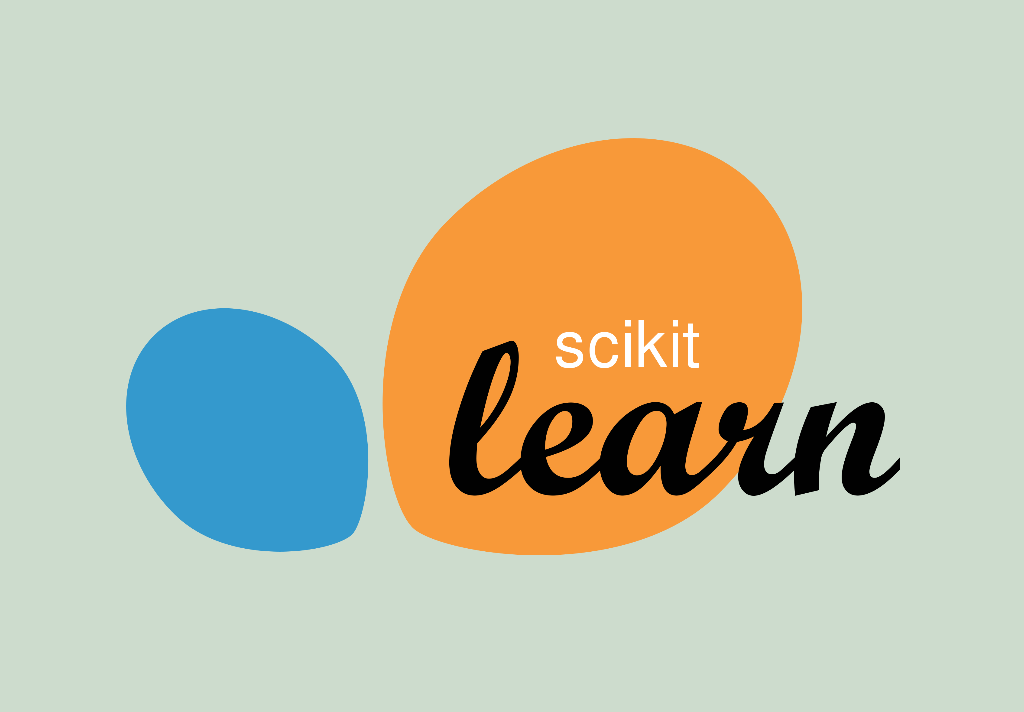
scikit-learn Machine Learning XGBoost
사이킷런 머신러닝 XGBoost
import pandas as pd
import numpy as np
import matplotlib.pyplot as plt
import seaborn as sns
Data Collection¶
# https://www.kaggle.com/datasets/annavictoria/speed-dating-experiment
file_url = 'https://raw.githubusercontent.com/dev-EthanJ/scikit-learn_Machine_Learning/main/data/'
file_name = 'dating.csv'
df = pd.read_csv(file_url + file_name)
df.head()
has_null | gender | age | age_o | race | race_o | importance_same_race | importance_same_religion | pref_o_attractive | pref_o_sincere | ... | funny_partner | ambition_partner | shared_interests_partner | interests_correlate | expected_happy_with_sd_people | expected_num_interested_in_me | like | guess_prob_liked | met | match | |
---|---|---|---|---|---|---|---|---|---|---|---|---|---|---|---|---|---|---|---|---|---|
0 | 0 | female | 21.0 | 27.0 | Asian/PacificIslander/Asian-American | European/Caucasian-American | 2.0 | 4.0 | 35.0 | 20.0 | ... | 7.0 | 6.0 | 5.0 | 0.14 | 3.0 | 2.0 | 7.0 | 6.0 | 0.0 | 0 |
1 | 0 | female | 21.0 | 22.0 | Asian/PacificIslander/Asian-American | European/Caucasian-American | 2.0 | 4.0 | 60.0 | 0.0 | ... | 8.0 | 5.0 | 6.0 | 0.54 | 3.0 | 2.0 | 7.0 | 5.0 | 1.0 | 0 |
2 | 1 | female | 21.0 | 22.0 | Asian/PacificIslander/Asian-American | Asian/PacificIslander/Asian-American | 2.0 | 4.0 | 19.0 | 18.0 | ... | 8.0 | 5.0 | 7.0 | 0.16 | 3.0 | 2.0 | 7.0 | NaN | 1.0 | 1 |
3 | 0 | female | 21.0 | 23.0 | Asian/PacificIslander/Asian-American | European/Caucasian-American | 2.0 | 4.0 | 30.0 | 5.0 | ... | 7.0 | 6.0 | 8.0 | 0.61 | 3.0 | 2.0 | 7.0 | 6.0 | 0.0 | 1 |
4 | 0 | female | 21.0 | 24.0 | Asian/PacificIslander/Asian-American | Latino/HispanicAmerican | 2.0 | 4.0 | 30.0 | 10.0 | ... | 7.0 | 6.0 | 6.0 | 0.21 | 3.0 | 2.0 | 6.0 | 6.0 | 0.0 | 1 |
5 rows × 39 columns
pd.options.display.max_columns = len(df.columns)
df.head()
has_null | gender | age | age_o | race | race_o | importance_same_race | importance_same_religion | pref_o_attractive | pref_o_sincere | pref_o_intelligence | pref_o_funny | pref_o_ambitious | pref_o_shared_interests | attractive_o | sincere_o | intelligence_o | funny_o | ambitous_o | shared_interests_o | attractive_important | sincere_important | intellicence_important | funny_important | ambtition_important | shared_interests_important | attractive_partner | sincere_partner | intelligence_partner | funny_partner | ambition_partner | shared_interests_partner | interests_correlate | expected_happy_with_sd_people | expected_num_interested_in_me | like | guess_prob_liked | met | match | |
---|---|---|---|---|---|---|---|---|---|---|---|---|---|---|---|---|---|---|---|---|---|---|---|---|---|---|---|---|---|---|---|---|---|---|---|---|---|---|---|
0 | 0 | female | 21.0 | 27.0 | Asian/PacificIslander/Asian-American | European/Caucasian-American | 2.0 | 4.0 | 35.0 | 20.0 | 20.0 | 20.0 | 0.0 | 5.0 | 6.0 | 8.0 | 8.0 | 8.0 | 8.0 | 6.0 | 15.0 | 20.0 | 20.0 | 15.0 | 15.0 | 15.0 | 6.0 | 9.0 | 7.0 | 7.0 | 6.0 | 5.0 | 0.14 | 3.0 | 2.0 | 7.0 | 6.0 | 0.0 | 0 |
1 | 0 | female | 21.0 | 22.0 | Asian/PacificIslander/Asian-American | European/Caucasian-American | 2.0 | 4.0 | 60.0 | 0.0 | 0.0 | 40.0 | 0.0 | 0.0 | 7.0 | 8.0 | 10.0 | 7.0 | 7.0 | 5.0 | 15.0 | 20.0 | 20.0 | 15.0 | 15.0 | 15.0 | 7.0 | 8.0 | 7.0 | 8.0 | 5.0 | 6.0 | 0.54 | 3.0 | 2.0 | 7.0 | 5.0 | 1.0 | 0 |
2 | 1 | female | 21.0 | 22.0 | Asian/PacificIslander/Asian-American | Asian/PacificIslander/Asian-American | 2.0 | 4.0 | 19.0 | 18.0 | 19.0 | 18.0 | 14.0 | 12.0 | 10.0 | 10.0 | 10.0 | 10.0 | 10.0 | 10.0 | 15.0 | 20.0 | 20.0 | 15.0 | 15.0 | 15.0 | 5.0 | 8.0 | 9.0 | 8.0 | 5.0 | 7.0 | 0.16 | 3.0 | 2.0 | 7.0 | NaN | 1.0 | 1 |
3 | 0 | female | 21.0 | 23.0 | Asian/PacificIslander/Asian-American | European/Caucasian-American | 2.0 | 4.0 | 30.0 | 5.0 | 15.0 | 40.0 | 5.0 | 5.0 | 7.0 | 8.0 | 9.0 | 8.0 | 9.0 | 8.0 | 15.0 | 20.0 | 20.0 | 15.0 | 15.0 | 15.0 | 7.0 | 6.0 | 8.0 | 7.0 | 6.0 | 8.0 | 0.61 | 3.0 | 2.0 | 7.0 | 6.0 | 0.0 | 1 |
4 | 0 | female | 21.0 | 24.0 | Asian/PacificIslander/Asian-American | Latino/HispanicAmerican | 2.0 | 4.0 | 30.0 | 10.0 | 20.0 | 10.0 | 10.0 | 20.0 | 8.0 | 7.0 | 9.0 | 6.0 | 9.0 | 7.0 | 15.0 | 20.0 | 20.0 | 15.0 | 15.0 | 15.0 | 5.0 | 6.0 | 7.0 | 7.0 | 6.0 | 6.0 | 0.21 | 3.0 | 2.0 | 6.0 | 6.0 | 0.0 | 1 |
df.info()
<class 'pandas.core.frame.DataFrame'> RangeIndex: 8378 entries, 0 to 8377 Data columns (total 39 columns): # Column Non-Null Count Dtype --- ------ -------------- ----- 0 has_null 8378 non-null int64 1 gender 8378 non-null object 2 age 8283 non-null float64 3 age_o 8274 non-null float64 4 race 8315 non-null object 5 race_o 8305 non-null object 6 importance_same_race 8299 non-null float64 7 importance_same_religion 8299 non-null float64 8 pref_o_attractive 8289 non-null float64 9 pref_o_sincere 8289 non-null float64 10 pref_o_intelligence 8289 non-null float64 11 pref_o_funny 8280 non-null float64 12 pref_o_ambitious 8271 non-null float64 13 pref_o_shared_interests 8249 non-null float64 14 attractive_o 8166 non-null float64 15 sincere_o 8091 non-null float64 16 intelligence_o 8072 non-null float64 17 funny_o 8018 non-null float64 18 ambitous_o 7656 non-null float64 19 shared_interests_o 7302 non-null float64 20 attractive_important 8299 non-null float64 21 sincere_important 8299 non-null float64 22 intellicence_important 8299 non-null float64 23 funny_important 8289 non-null float64 24 ambtition_important 8279 non-null float64 25 shared_interests_important 8257 non-null float64 26 attractive_partner 8176 non-null float64 27 sincere_partner 8101 non-null float64 28 intelligence_partner 8082 non-null float64 29 funny_partner 8028 non-null float64 30 ambition_partner 7666 non-null float64 31 shared_interests_partner 7311 non-null float64 32 interests_correlate 8220 non-null float64 33 expected_happy_with_sd_people 8277 non-null float64 34 expected_num_interested_in_me 1800 non-null float64 35 like 8138 non-null float64 36 guess_prob_liked 8069 non-null float64 37 met 8003 non-null float64 38 match 8378 non-null int64 dtypes: float64(34), int64(2), object(3) memory usage: 2.5+ MB
변수 목록¶
has_null
- 변수 중 Null값이 있는지 여부. 단, 이 데이터는 기존 데이터에서 일부 변수들이 생략된 축소판이기 때문에, 여기서 보이는 Null값 여부와 다소 차이가 있을 수 있음.
- 전반적으로 무응답 항목이 있는지에 대한 정보이므로 그대로 사용
age
/age_o
: age는 본인 나이이며 age_o는 상대방 나이.race
/race_o
: 마찬가지로 본인과 상대의 인종 정보.importance_same_race
/importance_same_religion
- 인종과 종교를 중요시 여기는지에 대한 응답
attractive(매력적인), sincere(성실한), intelligence(지적), funny(재미난), ambitious(야심찬), shared_ interests(공통관심사)
이 항목들은 4가지 관점에서 평가되어 총 변수가 24(6 × 4)개pref_o_xxx
( 예 : pref_o_attractive) : 상대방이 xxx 항목을 얼마나 중요하게 생각하는가에 대한 응답xxx_o
(예: attractive_o) : 상대방이 본인에 대한 xxx 항목을 평가한 항목xxx_important
(예 : attractive_important) : xxx 항목에 대해 본인이 얼마나 중요하게 생각하는가에 대한 응답xxx_partner
(예 : attractive_partner) : 본인이 상대방에 대한 xxx 항목을 평가한 항목
interests_correlate
: 관심사(취미 등) 연관도expected_happy_with_sd_people
: 스피드 데이팅을 통해 만난 사람과 함께할 때 얼마나 좋을 지에 대한 기대치expected_num_interested_in_me
: 얼마나 많은 사람이 나에게 관심을 보일지에 대한 기대치like
: 파트너가 마음에 들었는지 여부guess_prob_liked
: 파트너가 나를 마음에 들어했을지에 대한 예상met
: 파트너를 스피드 데이팅 이벤트 이전에 만난 적이 있는지 여부
pd.options.display.float_format = '{:.2f}'.format
df.describe()
has_null | age | age_o | importance_same_race | importance_same_religion | pref_o_attractive | pref_o_sincere | pref_o_intelligence | pref_o_funny | pref_o_ambitious | pref_o_shared_interests | attractive_o | sincere_o | intelligence_o | funny_o | ambitous_o | shared_interests_o | attractive_important | sincere_important | intellicence_important | funny_important | ambtition_important | shared_interests_important | attractive_partner | sincere_partner | intelligence_partner | funny_partner | ambition_partner | shared_interests_partner | interests_correlate | expected_happy_with_sd_people | expected_num_interested_in_me | like | guess_prob_liked | met | match | |
---|---|---|---|---|---|---|---|---|---|---|---|---|---|---|---|---|---|---|---|---|---|---|---|---|---|---|---|---|---|---|---|---|---|---|---|---|
count | 8378.00 | 8283.00 | 8274.00 | 8299.00 | 8299.00 | 8289.00 | 8289.00 | 8289.00 | 8280.00 | 8271.00 | 8249.00 | 8166.00 | 8091.00 | 8072.00 | 8018.00 | 7656.00 | 7302.00 | 8299.00 | 8299.00 | 8299.00 | 8289.00 | 8279.00 | 8257.00 | 8176.00 | 8101.00 | 8082.00 | 8028.00 | 7666.00 | 7311.00 | 8220.00 | 8277.00 | 1800.00 | 8138.00 | 8069.00 | 8003.00 | 8378.00 |
mean | 0.87 | 26.36 | 26.36 | 3.78 | 3.65 | 22.50 | 17.40 | 20.27 | 17.46 | 10.69 | 11.85 | 6.19 | 7.18 | 7.37 | 6.40 | 6.78 | 5.47 | 22.51 | 17.40 | 20.27 | 17.46 | 10.68 | 11.85 | 6.19 | 7.18 | 7.37 | 6.40 | 6.78 | 5.47 | 0.20 | 5.53 | 5.57 | 6.13 | 5.21 | 0.05 | 0.16 |
std | 0.33 | 3.57 | 3.56 | 2.85 | 2.81 | 12.57 | 7.04 | 6.78 | 6.09 | 6.13 | 6.36 | 1.95 | 1.74 | 1.55 | 1.95 | 1.79 | 2.16 | 12.59 | 7.05 | 6.78 | 6.09 | 6.12 | 6.36 | 1.95 | 1.74 | 1.55 | 1.95 | 1.79 | 2.16 | 0.30 | 1.73 | 4.76 | 1.84 | 2.13 | 0.28 | 0.37 |
min | 0.00 | 18.00 | 18.00 | 0.00 | 1.00 | 0.00 | 0.00 | 0.00 | 0.00 | 0.00 | 0.00 | 0.00 | 0.00 | 0.00 | 0.00 | 0.00 | 0.00 | 0.00 | 0.00 | 0.00 | 0.00 | 0.00 | 0.00 | 0.00 | 0.00 | 0.00 | 0.00 | 0.00 | 0.00 | -0.83 | 1.00 | 0.00 | 0.00 | 0.00 | 0.00 | 0.00 |
25% | 1.00 | 24.00 | 24.00 | 1.00 | 1.00 | 15.00 | 15.00 | 17.39 | 15.00 | 5.00 | 9.52 | 5.00 | 6.00 | 6.00 | 5.00 | 6.00 | 4.00 | 15.00 | 15.00 | 17.39 | 15.00 | 5.00 | 9.52 | 5.00 | 6.00 | 6.00 | 5.00 | 6.00 | 4.00 | -0.02 | 5.00 | 2.00 | 5.00 | 4.00 | 0.00 | 0.00 |
50% | 1.00 | 26.00 | 26.00 | 3.00 | 3.00 | 20.00 | 18.37 | 20.00 | 18.00 | 10.00 | 10.64 | 6.00 | 7.00 | 7.00 | 7.00 | 7.00 | 6.00 | 20.00 | 18.18 | 20.00 | 18.00 | 10.00 | 10.64 | 6.00 | 7.00 | 7.00 | 7.00 | 7.00 | 6.00 | 0.21 | 6.00 | 4.00 | 6.00 | 5.00 | 0.00 | 0.00 |
75% | 1.00 | 28.00 | 28.00 | 6.00 | 6.00 | 25.00 | 20.00 | 23.81 | 20.00 | 15.00 | 16.00 | 8.00 | 8.00 | 8.00 | 8.00 | 8.00 | 7.00 | 25.00 | 20.00 | 23.81 | 20.00 | 15.00 | 16.00 | 8.00 | 8.00 | 8.00 | 8.00 | 8.00 | 7.00 | 0.43 | 7.00 | 8.00 | 7.00 | 7.00 | 0.00 | 0.00 |
max | 1.00 | 55.00 | 55.00 | 10.00 | 10.00 | 100.00 | 60.00 | 50.00 | 50.00 | 53.00 | 30.00 | 10.50 | 10.00 | 10.00 | 11.00 | 10.00 | 10.00 | 100.00 | 60.00 | 50.00 | 50.00 | 53.00 | 30.00 | 10.00 | 10.00 | 10.00 | 10.00 | 10.00 | 10.00 | 0.91 | 10.00 | 20.00 | 10.00 | 10.00 | 8.00 | 1.00 |
Data pre-processing¶
missing value¶
df.isna().mean().sort_values(ascending=False)
expected_num_interested_in_me 0.79 shared_interests_o 0.13 shared_interests_partner 0.13 ambitous_o 0.09 ambition_partner 0.08 met 0.04 funny_o 0.04 funny_partner 0.04 guess_prob_liked 0.04 intelligence_o 0.04 intelligence_partner 0.04 sincere_o 0.03 sincere_partner 0.03 like 0.03 attractive_o 0.03 attractive_partner 0.02 interests_correlate 0.02 pref_o_shared_interests 0.02 shared_interests_important 0.01 pref_o_ambitious 0.01 age_o 0.01 expected_happy_with_sd_people 0.01 ambtition_important 0.01 pref_o_funny 0.01 age 0.01 pref_o_sincere 0.01 pref_o_attractive 0.01 funny_important 0.01 pref_o_intelligence 0.01 attractive_important 0.01 intellicence_important 0.01 sincere_important 0.01 importance_same_religion 0.01 importance_same_race 0.01 race_o 0.01 race 0.01 has_null 0.00 gender 0.00 match 0.00 dtype: float64
for col in df.columns:
if df[col].isna().mean() < 0.02:
df = df.dropna(subset=[col])
df.isna().sum()
has_null 0 gender 0 age 0 age_o 0 race 0 race_o 0 importance_same_race 0 importance_same_religion 0 pref_o_attractive 0 pref_o_sincere 0 pref_o_intelligence 0 pref_o_funny 0 pref_o_ambitious 0 pref_o_shared_interests 0 attractive_o 176 sincere_o 247 intelligence_o 268 funny_o 321 ambitous_o 678 shared_interests_o 1029 attractive_important 0 sincere_important 0 intellicence_important 0 funny_important 0 ambtition_important 0 shared_interests_important 0 attractive_partner 176 sincere_partner 247 intelligence_partner 268 funny_partner 321 ambition_partner 678 shared_interests_partner 1029 interests_correlate 0 expected_happy_with_sd_people 0 expected_num_interested_in_me 6366 like 213 guess_prob_liked 278 met 343 match 0 dtype: int64
missing_columns = list()
for col in df.columns:
if df[col].isna().sum() > 0:
missing_columns.append(col)
missing_columns
['attractive_o', 'sincere_o', 'intelligence_o', 'funny_o', 'ambitous_o', 'shared_interests_o', 'attractive_partner', 'sincere_partner', 'intelligence_partner', 'funny_partner', 'ambition_partner', 'shared_interests_partner', 'expected_num_interested_in_me', 'like', 'guess_prob_liked', 'met']
df = df.fillna(-99)
df.info()
<class 'pandas.core.frame.DataFrame'> Int64Index: 8086 entries, 0 to 8377 Data columns (total 39 columns): # Column Non-Null Count Dtype --- ------ -------------- ----- 0 has_null 8086 non-null int64 1 gender 8086 non-null object 2 age 8086 non-null float64 3 age_o 8086 non-null float64 4 race 8086 non-null object 5 race_o 8086 non-null object 6 importance_same_race 8086 non-null float64 7 importance_same_religion 8086 non-null float64 8 pref_o_attractive 8086 non-null float64 9 pref_o_sincere 8086 non-null float64 10 pref_o_intelligence 8086 non-null float64 11 pref_o_funny 8086 non-null float64 12 pref_o_ambitious 8086 non-null float64 13 pref_o_shared_interests 8086 non-null float64 14 attractive_o 8086 non-null float64 15 sincere_o 8086 non-null float64 16 intelligence_o 8086 non-null float64 17 funny_o 8086 non-null float64 18 ambitous_o 8086 non-null float64 19 shared_interests_o 8086 non-null float64 20 attractive_important 8086 non-null float64 21 sincere_important 8086 non-null float64 22 intellicence_important 8086 non-null float64 23 funny_important 8086 non-null float64 24 ambtition_important 8086 non-null float64 25 shared_interests_important 8086 non-null float64 26 attractive_partner 8086 non-null float64 27 sincere_partner 8086 non-null float64 28 intelligence_partner 8086 non-null float64 29 funny_partner 8086 non-null float64 30 ambition_partner 8086 non-null float64 31 shared_interests_partner 8086 non-null float64 32 interests_correlate 8086 non-null float64 33 expected_happy_with_sd_people 8086 non-null float64 34 expected_num_interested_in_me 8086 non-null float64 35 like 8086 non-null float64 36 guess_prob_liked 8086 non-null float64 37 met 8086 non-null float64 38 match 8086 non-null int64 dtypes: float64(34), int64(2), object(3) memory usage: 2.5+ MB
missing_columns
['attractive_o', 'sincere_o', 'intelligence_o', 'funny_o', 'ambitous_o', 'shared_interests_o', 'attractive_partner', 'sincere_partner', 'intelligence_partner', 'funny_partner', 'ambition_partner', 'shared_interests_partner', 'expected_num_interested_in_me', 'like', 'guess_prob_liked', 'met']
Feature Engineering¶
- Feature: 독립변수들 + Engineering: 가공
age_gap
¶
age
: 본인의 나이age_o
: 상대방의 나이
def age_gap(row):
# female: (age_o - age)
if row['gender'] == 'female':
return row['age_o'] - row['age']
# male: (age - age_o)
if row['gender'] == 'male':
return row['age'] - row['age_o']
df['age_gap'] = df.apply(age_gap, axis=1)
df['age_gap'].head(10)
0 6.00 1 1.00 2 1.00 3 2.00 4 3.00 5 4.00 6 9.00 7 6.00 8 7.00 9 3.00 Name: age_gap, dtype: float64
- 나이 차이의 절대값 column 생성
df['age_gap_abs'] = abs(df['age_gap'])
df['age_gap_abs'].unique()
array([ 6., 1., 2., 3., 4., 9., 7., 0., 5., 8., 12., 13., 10., 17., 16., 11., 14., 18., 15., 19., 20., 22., 28., 32.])
same_race
¶
def check_same_race(record):
if record['race'] == record['race_o']:
return 1
else:
return -1
df['same_race'] = df.apply(check_same_race, axis=1)
df['same_race'].unique()
array([-1, 1], dtype=int64)
df['importance_same_race'].value_counts()
1.00 2721 3.00 962 2.00 935 5.00 640 8.00 629 7.00 533 6.00 515 4.00 494 9.00 403 10.00 246 0.00 8 Name: importance_same_race, dtype: int64
df['same_race'].isna().sum()
0
'importance_same_race' in missing_columns
False
same_race_point
¶
same_race_point
=same_race
*importance_same_race
- 파생변수
same_race_point
- 파생변수
df['same_race_point'] = df['same_race'].mul(df['importance_same_race'])
df['same_race_point'].value_counts()
-1.00 1755 1.00 966 -3.00 610 -2.00 573 2.00 362 -5.00 353 3.00 352 8.00 320 -6.00 316 -7.00 310 -8.00 309 5.00 287 -4.00 272 7.00 223 4.00 222 -9.00 210 6.00 199 9.00 193 10.00 130 -10.00 116 0.00 8 Name: same_race_point, dtype: int64
df[['race', 'race_o', 'same_race', 'importance_same_race', 'same_race_point']].head(10)
race | race_o | same_race | importance_same_race | same_race_point | |
---|---|---|---|---|---|
0 | Asian/PacificIslander/Asian-American | European/Caucasian-American | -1 | 2.00 | -2.00 |
1 | Asian/PacificIslander/Asian-American | European/Caucasian-American | -1 | 2.00 | -2.00 |
2 | Asian/PacificIslander/Asian-American | Asian/PacificIslander/Asian-American | 1 | 2.00 | 2.00 |
3 | Asian/PacificIslander/Asian-American | European/Caucasian-American | -1 | 2.00 | -2.00 |
4 | Asian/PacificIslander/Asian-American | Latino/HispanicAmerican | -1 | 2.00 | -2.00 |
5 | Asian/PacificIslander/Asian-American | European/Caucasian-American | -1 | 2.00 | -2.00 |
6 | Asian/PacificIslander/Asian-American | European/Caucasian-American | -1 | 2.00 | -2.00 |
7 | Asian/PacificIslander/Asian-American | European/Caucasian-American | -1 | 2.00 | -2.00 |
8 | Asian/PacificIslander/Asian-American | European/Caucasian-American | -1 | 2.00 | -2.00 |
9 | Asian/PacificIslander/Asian-American | European/Caucasian-American | -1 | 2.00 | -2.00 |
rating
¶
importance
*score
=rate
: 파생변수
def rating(record, importance, score):
# 결측치 처리
if record[importance] == -99: return None
if record[score] == -99: return None
return record[importance] * record[score]
df.columns
Index(['has_null', 'gender', 'age', 'age_o', 'race', 'race_o', 'importance_same_race', 'importance_same_religion', 'pref_o_attractive', 'pref_o_sincere', 'pref_o_intelligence', 'pref_o_funny', 'pref_o_ambitious', 'pref_o_shared_interests', 'attractive_o', 'sincere_o', 'intelligence_o', 'funny_o', 'ambitous_o', 'shared_interests_o', 'attractive_important', 'sincere_important', 'intellicence_important', 'funny_important', 'ambtition_important', 'shared_interests_important', 'attractive_partner', 'sincere_partner', 'intelligence_partner', 'funny_partner', 'ambition_partner', 'shared_interests_partner', 'interests_correlate', 'expected_happy_with_sd_people', 'expected_num_interested_in_me', 'like', 'guess_prob_liked', 'met', 'match', 'age_gap', 'age_gap_abs', 'same_race', 'same_race_point'], dtype='object')
- 특성에 대한 상대방의 선호도
pref_ptnr = [col for col in df.columns if col.split('_')[0] == 'pref']
pref_ptnr
['pref_o_attractive', 'pref_o_sincere', 'pref_o_intelligence', 'pref_o_funny', 'pref_o_ambitious', 'pref_o_shared_interests']
- (본인의) 특성에 대한 상대방의 평가
rate_me = [col for col in df.columns if col[-1] == 'o']
rate_me
['age_o', 'race_o', 'attractive_o', 'sincere_o', 'intelligence_o', 'funny_o', 'ambitous_o', 'shared_interests_o']
del rate_me[0:2]
rate_me
['attractive_o', 'sincere_o', 'intelligence_o', 'funny_o', 'ambitous_o', 'shared_interests_o']
- 특성에 대한 본인의 선호도
pref_me = [col for col in df.columns if col[-9:] == 'important']
pref_me
['attractive_important', 'sincere_important', 'intellicence_important', 'funny_important', 'ambtition_important', 'shared_interests_important']
- (상대방의) 특성에 대한 본인의 평가
rate_ptnr = [col for col in df.columns if col[-(len('partner')):] == 'partner']
rate_ptnr
['attractive_partner', 'sincere_partner', 'intelligence_partner', 'funny_partner', 'ambition_partner', 'shared_interests_partner']
- 평가 점수 X 중요도 = 새로운 label:
score
character_list = [ch.split('_')[0] for ch in rate_ptnr]
score_ptnr = [ch + '_ptnr' for ch in character_list]
score_me = [ch + '_me' for ch in character_list]
print(score_ptnr)
print(score_me)
['attractive_ptnr', 'sincere_ptnr', 'intelligence_ptnr', 'funny_ptnr', 'ambition_ptnr', 'shared_ptnr'] ['attractive_me', 'sincere_me', 'intelligence_me', 'funny_me', 'ambition_me', 'shared_me']
for i, j, k in zip(score_ptnr, pref_ptnr, rate_ptnr):
df[i] = df.apply(lambda x: rating(x, j, k), axis=1)
for score in score_ptnr:
print(df[score].head())
0 210.00 1 420.00 2 95.00 3 210.00 4 150.00 Name: attractive_ptnr, dtype: float64 0 180.00 1 0.00 2 144.00 3 30.00 4 60.00 Name: sincere_ptnr, dtype: float64 0 140.00 1 0.00 2 171.00 3 120.00 4 140.00 Name: intelligence_ptnr, dtype: float64 0 140.00 1 320.00 2 144.00 3 280.00 4 70.00 Name: funny_ptnr, dtype: float64 0 0.00 1 0.00 2 70.00 3 30.00 4 60.00 Name: ambition_ptnr, dtype: float64 0 25.00 1 0.00 2 84.00 3 40.00 4 120.00 Name: shared_ptnr, dtype: float64
for i, j, k in zip(score_me, pref_me, rate_me):
df[i] = df.apply(lambda x: rating(x, j, k), axis=1)
for score in score_me:
print(df[score].head())
0 90.00 1 105.00 2 150.00 3 105.00 4 120.00 Name: attractive_me, dtype: float64 0 160.00 1 160.00 2 200.00 3 160.00 4 140.00 Name: sincere_me, dtype: float64 0 160.00 1 200.00 2 200.00 3 180.00 4 180.00 Name: intelligence_me, dtype: float64 0 120.00 1 105.00 2 150.00 3 120.00 4 90.00 Name: funny_me, dtype: float64 0 120.00 1 105.00 2 150.00 3 135.00 4 135.00 Name: ambition_me, dtype: float64 0 90.00 1 75.00 2 150.00 3 120.00 4 105.00 Name: shared_me, dtype: float64
df.columns
Index(['has_null', 'gender', 'age', 'age_o', 'race', 'race_o', 'importance_same_race', 'importance_same_religion', 'pref_o_attractive', 'pref_o_sincere', 'pref_o_intelligence', 'pref_o_funny', 'pref_o_ambitious', 'pref_o_shared_interests', 'attractive_o', 'sincere_o', 'intelligence_o', 'funny_o', 'ambitous_o', 'shared_interests_o', 'attractive_important', 'sincere_important', 'intellicence_important', 'funny_important', 'ambtition_important', 'shared_interests_important', 'attractive_partner', 'sincere_partner', 'intelligence_partner', 'funny_partner', 'ambition_partner', 'shared_interests_partner', 'interests_correlate', 'expected_happy_with_sd_people', 'expected_num_interested_in_me', 'like', 'guess_prob_liked', 'met', 'match', 'age_gap', 'age_gap_abs', 'same_race', 'same_race_point', 'attractive_ptnr', 'sincere_ptnr', 'intelligence_ptnr', 'funny_ptnr', 'ambition_ptnr', 'shared_ptnr', 'attractive_me', 'sincere_me', 'intelligence_me', 'funny_me', 'ambition_me', 'shared_me'], dtype='object')
Categorical Data¶
df.info()
<class 'pandas.core.frame.DataFrame'> Int64Index: 8086 entries, 0 to 8377 Data columns (total 55 columns): # Column Non-Null Count Dtype --- ------ -------------- ----- 0 has_null 8086 non-null int64 1 gender 8086 non-null object 2 age 8086 non-null float64 3 age_o 8086 non-null float64 4 race 8086 non-null object 5 race_o 8086 non-null object 6 importance_same_race 8086 non-null float64 7 importance_same_religion 8086 non-null float64 8 pref_o_attractive 8086 non-null float64 9 pref_o_sincere 8086 non-null float64 10 pref_o_intelligence 8086 non-null float64 11 pref_o_funny 8086 non-null float64 12 pref_o_ambitious 8086 non-null float64 13 pref_o_shared_interests 8086 non-null float64 14 attractive_o 8086 non-null float64 15 sincere_o 8086 non-null float64 16 intelligence_o 8086 non-null float64 17 funny_o 8086 non-null float64 18 ambitous_o 8086 non-null float64 19 shared_interests_o 8086 non-null float64 20 attractive_important 8086 non-null float64 21 sincere_important 8086 non-null float64 22 intellicence_important 8086 non-null float64 23 funny_important 8086 non-null float64 24 ambtition_important 8086 non-null float64 25 shared_interests_important 8086 non-null float64 26 attractive_partner 8086 non-null float64 27 sincere_partner 8086 non-null float64 28 intelligence_partner 8086 non-null float64 29 funny_partner 8086 non-null float64 30 ambition_partner 8086 non-null float64 31 shared_interests_partner 8086 non-null float64 32 interests_correlate 8086 non-null float64 33 expected_happy_with_sd_people 8086 non-null float64 34 expected_num_interested_in_me 8086 non-null float64 35 like 8086 non-null float64 36 guess_prob_liked 8086 non-null float64 37 met 8086 non-null float64 38 match 8086 non-null int64 39 age_gap 8086 non-null float64 40 age_gap_abs 8086 non-null float64 41 same_race 8086 non-null int64 42 same_race_point 8086 non-null float64 43 attractive_ptnr 7910 non-null float64 44 sincere_ptnr 7839 non-null float64 45 intelligence_ptnr 7818 non-null float64 46 funny_ptnr 7765 non-null float64 47 ambition_ptnr 7408 non-null float64 48 shared_ptnr 7057 non-null float64 49 attractive_me 7910 non-null float64 50 sincere_me 7839 non-null float64 51 intelligence_me 7818 non-null float64 52 funny_me 7765 non-null float64 53 ambition_me 7408 non-null float64 54 shared_me 7057 non-null float64 dtypes: float64(49), int64(3), object(3) memory usage: 3.5+ MB
df.describe(include=['O'])
gender | race | race_o | |
---|---|---|---|
count | 8086 | 8086 | 8086 |
unique | 2 | 5 | 5 |
top | female | European/Caucasian-American | European/Caucasian-American |
freq | 4043 | 4594 | 4594 |
df = pd.get_dummies(df, columns=['gender', 'race', 'race_o'], drop_first=True)
df.info()
<class 'pandas.core.frame.DataFrame'> Int64Index: 8086 entries, 0 to 8377 Data columns (total 61 columns): # Column Non-Null Count Dtype --- ------ -------------- ----- 0 has_null 8086 non-null int64 1 age 8086 non-null float64 2 age_o 8086 non-null float64 3 importance_same_race 8086 non-null float64 4 importance_same_religion 8086 non-null float64 5 pref_o_attractive 8086 non-null float64 6 pref_o_sincere 8086 non-null float64 7 pref_o_intelligence 8086 non-null float64 8 pref_o_funny 8086 non-null float64 9 pref_o_ambitious 8086 non-null float64 10 pref_o_shared_interests 8086 non-null float64 11 attractive_o 8086 non-null float64 12 sincere_o 8086 non-null float64 13 intelligence_o 8086 non-null float64 14 funny_o 8086 non-null float64 15 ambitous_o 8086 non-null float64 16 shared_interests_o 8086 non-null float64 17 attractive_important 8086 non-null float64 18 sincere_important 8086 non-null float64 19 intellicence_important 8086 non-null float64 20 funny_important 8086 non-null float64 21 ambtition_important 8086 non-null float64 22 shared_interests_important 8086 non-null float64 23 attractive_partner 8086 non-null float64 24 sincere_partner 8086 non-null float64 25 intelligence_partner 8086 non-null float64 26 funny_partner 8086 non-null float64 27 ambition_partner 8086 non-null float64 28 shared_interests_partner 8086 non-null float64 29 interests_correlate 8086 non-null float64 30 expected_happy_with_sd_people 8086 non-null float64 31 expected_num_interested_in_me 8086 non-null float64 32 like 8086 non-null float64 33 guess_prob_liked 8086 non-null float64 34 met 8086 non-null float64 35 match 8086 non-null int64 36 age_gap 8086 non-null float64 37 age_gap_abs 8086 non-null float64 38 same_race 8086 non-null int64 39 same_race_point 8086 non-null float64 40 attractive_ptnr 7910 non-null float64 41 sincere_ptnr 7839 non-null float64 42 intelligence_ptnr 7818 non-null float64 43 funny_ptnr 7765 non-null float64 44 ambition_ptnr 7408 non-null float64 45 shared_ptnr 7057 non-null float64 46 attractive_me 7910 non-null float64 47 sincere_me 7839 non-null float64 48 intelligence_me 7818 non-null float64 49 funny_me 7765 non-null float64 50 ambition_me 7408 non-null float64 51 shared_me 7057 non-null float64 52 gender_male 8086 non-null uint8 53 race_Black/AfricanAmerican 8086 non-null uint8 54 race_European/Caucasian-American 8086 non-null uint8 55 race_Latino/HispanicAmerican 8086 non-null uint8 56 race_Other 8086 non-null uint8 57 race_o_Black/AfricanAmerican 8086 non-null uint8 58 race_o_European/Caucasian-American 8086 non-null uint8 59 race_o_Latino/HispanicAmerican 8086 non-null uint8 60 race_o_Other 8086 non-null uint8 dtypes: float64(49), int64(3), uint8(9) memory usage: 3.3 MB
Training Model¶
train set, test set¶
from sklearn.model_selection import train_test_split
X = df.drop(columns=['match'])
y = df['match']
X_train, X_test, y_train, y_test = train_test_split(
X, y, test_size=0.25, random_state=814
)
Modeling¶
import xgboost as xgb
model = xgb.XGBClassifier(n_estimators=500, max_depth=5, random_state=np.random.randint(1000))
model.fit(X_train, y_train)
pred = model.predict(X_test)
pred
C:\Users\EthanJ\anaconda3\lib\site-packages\xgboost\sklearn.py:1224: UserWarning: The use of label encoder in XGBClassifier is deprecated and will be removed in a future release. To remove this warning, do the following: 1) Pass option use_label_encoder=False when constructing XGBClassifier object; and 2) Encode your labels (y) as integers starting with 0, i.e. 0, 1, 2, ..., [num_class - 1]. warnings.warn(label_encoder_deprecation_msg, UserWarning) C:\Users\EthanJ\anaconda3\lib\site-packages\xgboost\data.py:250: FutureWarning: pandas.Int64Index is deprecated and will be removed from pandas in a future version. Use pandas.Index with the appropriate dtype instead. elif isinstance(data.columns, (pd.Int64Index, pd.RangeIndex)):
[17:56:14] WARNING: C:\Windows\Temp\abs_557yfx631l\croots\recipe\xgboost-split_1659548953302\work\src\learner.cc:1115: Starting in XGBoost 1.3.0, the default evaluation metric used with the objective 'binary:logistic' was changed from 'error' to 'logloss'. Explicitly set eval_metric if you'd like to restore the old behavior.
array([0, 0, 0, ..., 0, 1, 0], dtype=int64)
Evaluating Model¶
from sklearn.metrics import accuracy_score, confusion_matrix, classification_report
# 매칭 여부
match_mean = df['match'].mean()
print(match_mean)
# 데이터의 편향성
print(1 - match_mean)
print()
print(accuracy_score(y_test, pred))
0.16547118476378928 0.8345288152362107 0.8768545994065282
Confusion Matrix¶
- 1종 오류: Actual 0, Predicted 1 = False Positive(FP)
- 2종 오류: Actual 1, Predicted 0 = False Negative(FN)
print(confusion_matrix(y_test, pred))
[[1622 72] [ 177 151]]
cf_matrix = confusion_matrix(y_test, pred)
cf_matrix
array([[1622, 72], [ 177, 151]], dtype=int64)
group_name = ['TN', 'FP', 'FN', 'TP']
group_counts = ["{0:0.0f}".format(value) for value in cf_matrix.flatten()]
group_percent = ["{0:.2%}".format(value) for value in cf_matrix.flatten() / np.sum(cf_matrix)]
labels = [f"{name}\n{count}\n{percent}" for name, count, percent
in zip(group_name, group_counts, group_percent)]
labels = np.asarray(labels).reshape(2, 2)
sns.heatmap(cf_matrix, annot=labels, fmt='', cmap='coolwarm')
plt.ylabel('Actual')
plt.xlabel('Predicted')
plt.show()
classification_report(y_true, y_pred)
¶
종속변수(y)의 값인
0
과1
각각에 대해 나타남예측하려는 경우인
1
에 대한 값을 주로 해석한다.precision
: 정밀도recall
: 재현율f1-score
: F1-점수support
: index
print(classification_report(y_test, pred))
precision recall f1-score support 0 0.90 0.96 0.93 1694 1 0.68 0.46 0.55 328 accuracy 0.88 2022 macro avg 0.79 0.71 0.74 2022 weighted avg 0.87 0.88 0.87 2022
정밀도(precision)¶
- 1로 예측한 경우 중, 얼마만큼이 실제로 1인지를 나타냄
$TP\over(TP+FP)$=$\frac{TP}{Predicted True}$FP(1종 오류)가 커질수록 분모가 커지기 때문에 정밀도는 낮아짐
재현율(recall)¶
- 실제 1 중에, 얼마만큼을 1로 예측했는지 나타냄
$TP\over(TP+FN)$=$\frac{TP}{ActualTrue}$FN(2종 오류)가 커질수록 분모가 커지기 때문에 재현율은 낮아짐
F1-점수(f1-score)¶
정밀도와 재현율의 조화평균
- 조화평균 : 주어진 수들의 역수의 산술평균의 역수
$H = \frac{2a_1a_2}{a_1 + a_2}$
- 조화평균 : 주어진 수들의 역수의 산술평균의 역수
F1-score = $2 \times \frac{precision \times recall}{precesion + recall}$=$2 \times \frac{정밀도 \times 재현율}{정밀도 + 재현율}$
1종 오류가 중요하면
precision
, 2종 오류가 중요하면recall
딱히 중요한 것 없으면F1-score
Hyperparameter tuning¶
경사하강법(Gradient descent)¶
XGBoost 등에서 Machine Learning을 학습시킬 때 최소의 오차를 찾는 방법
오차 함수에 대한 경사도(미분계수, 기울기, Gradient)를 기준으로 매개변수를 반복해서 이동하며 오차의 최소값을 찾음
- 매개변수: 선형 회귀에서의 계수(변수에 대한 기울기 값)
경사하강법과 보폭¶
Gradient Boosting의 핵심개념, model이 최소 오차값을 만드는 매개변수들을 학습하는 방법론
오차식에 대한 미분계수를 통해 매개변수의 이동 방향과 보폭을 결정
- 보폭: 매개변수의 이동량
미분계수(기울기)¶
평균변화율에서 x의 증가량이 0에 무한히 가까워질 때 ($x → 0$) 평균변화율 값
그래프상에서의 접선의 기울기, 계수
GirdSearchCV¶
- 사용자가 직접 model의 hyperparameter의 값을 list로 입력하면 값에 대한 경우의 수마다
예측 성능을 측정 평가, 비교하면서 최적의 hyperparameter 값을 찾는 과정을 진행
Hyperparameter 종류¶
learning_rate
(학습률)Gradient descent에서 'parameter'를 얼만큼씩 이동하면서 오차 최소값을 찾을 지 보폭의 크기를 결정
기본적으로 보폭은 미분계수에 의해 결정되지만,
learning_rate
에 비례해서 보폭이 증감됨.학습률과 보폭:
learning_rate
= input → 보폭 = output과도하게 작은
learning_rate
: 상당한 시간 소모, overfitting 발생과도하게 큰
learning_rate
: 결여된 학습적절한 크기의 학습률을 사용해야 적당한 시간에 최소 오차 지점을 찾을 수 있음
max_depth
: 각 tree의 깊이 제한subsample
: 모델을 학습시킬 때 전체 데이터 대비 트리에 사용할 데이터 량의 값0.5면 데이터의 절반씩 랜덤 추출
overfitting을 방지하는 데 도움을 준다.
n_estimators
: 전체 tree의 개수
gsCV_parameter = {
'learning_rate': [0.01, 0.1, 0.2],
'max_depth': [3, 6, 9],
'subsample': [0.5, 0.75, 1],
'n_estimators': [250, 500, 1000]
}
from sklearn.model_selection import GridSearchCV
model = xgb.XGBClassifier()
gs_model = GridSearchCV(model, gsCV_parameter, n_jobs=6, scoring='f1', cv=5)
gs_model.fit(X_train, y_train)
C:\Users\EthanJ\anaconda3\lib\site-packages\xgboost\sklearn.py:1224: UserWarning: The use of label encoder in XGBClassifier is deprecated and will be removed in a future release. To remove this warning, do the following: 1) Pass option use_label_encoder=False when constructing XGBClassifier object; and 2) Encode your labels (y) as integers starting with 0, i.e. 0, 1, 2, ..., [num_class - 1]. warnings.warn(label_encoder_deprecation_msg, UserWarning) C:\Users\EthanJ\anaconda3\lib\site-packages\xgboost\data.py:250: FutureWarning: pandas.Int64Index is deprecated and will be removed from pandas in a future version. Use pandas.Index with the appropriate dtype instead. elif isinstance(data.columns, (pd.Int64Index, pd.RangeIndex)):
[17:49:37] WARNING: C:\Windows\Temp\abs_557yfx631l\croots\recipe\xgboost-split_1659548953302\work\src\learner.cc:1115: Starting in XGBoost 1.3.0, the default evaluation metric used with the objective 'binary:logistic' was changed from 'error' to 'logloss'. Explicitly set eval_metric if you'd like to restore the old behavior.
GridSearchCV(cv=5, estimator=XGBClassifier(base_score=None, booster=None, colsample_bylevel=None, colsample_bynode=None, colsample_bytree=None, enable_categorical=False, gamma=None, gpu_id=None, importance_type=None, interaction_constraints=None, learning_rate=None, max_delta_step=None, max_depth=None, min_child_weight=None, missing=nan, monotone_constraints=None, n_estimators=100, n_jobs=None, num_parallel_tree=None, predictor=None, random_state=None, reg_alpha=None, reg_lambda=None, scale_pos_weight=None, subsample=None, tree_method=None, validate_parameters=None, verbosity=None), n_jobs=6, param_grid={'learning_rate': [0.01, 0.1, 0.2], 'max_depth': [3, 6, 9], 'n_estimators': [250, 500, 1000], 'subsample': [0.5, 0.75, 1]}, scoring='f1')
import joblib
joblib.dump(gs_model, 'XGBoost_GridSearchCV_model.pkl')
['XGBoost_GridSearchCV_model.pkl']
- 가장 높은 정확도의 paramter set
gs_model.best_params_
{'learning_rate': 0.2, 'max_depth': 3, 'n_estimators': 1000, 'subsample': 0.75}
pred = gs_model.predict(X_test)
print("accuracy_score = ", accuracy_score(y_test, pred))
print(classification_report(y_test, pred))
accuracy_score = 0.8684470820969338 precision recall f1-score support 0 0.90 0.95 0.92 1694 1 0.63 0.45 0.53 328 accuracy 0.87 2022 macro avg 0.77 0.70 0.73 2022 weighted avg 0.86 0.87 0.86 2022
변수의 영향력: 중요 변수 확인¶
model = xgb.XGBClassifier(
learning_rate=0.3, max_depth=5, n_estimators=1000, subsample=0.5, random_state=100
)
model.fit(X_train, y_train)
[17:49:39] WARNING: C:\Windows\Temp\abs_557yfx631l\croots\recipe\xgboost-split_1659548953302\work\src\learner.cc:1115: Starting in XGBoost 1.3.0, the default evaluation metric used with the objective 'binary:logistic' was changed from 'error' to 'logloss'. Explicitly set eval_metric if you'd like to restore the old behavior.
C:\Users\EthanJ\anaconda3\lib\site-packages\xgboost\sklearn.py:1224: UserWarning: The use of label encoder in XGBClassifier is deprecated and will be removed in a future release. To remove this warning, do the following: 1) Pass option use_label_encoder=False when constructing XGBClassifier object; and 2) Encode your labels (y) as integers starting with 0, i.e. 0, 1, 2, ..., [num_class - 1]. warnings.warn(label_encoder_deprecation_msg, UserWarning) C:\Users\EthanJ\anaconda3\lib\site-packages\xgboost\data.py:250: FutureWarning: pandas.Int64Index is deprecated and will be removed from pandas in a future version. Use pandas.Index with the appropriate dtype instead. elif isinstance(data.columns, (pd.Int64Index, pd.RangeIndex)):
XGBClassifier(base_score=0.5, booster='gbtree', colsample_bylevel=1, colsample_bynode=1, colsample_bytree=1, enable_categorical=False, gamma=0, gpu_id=-1, importance_type=None, interaction_constraints='', learning_rate=0.3, max_delta_step=0, max_depth=5, min_child_weight=1, missing=nan, monotone_constraints='()', n_estimators=1000, n_jobs=16, num_parallel_tree=1, predictor='auto', random_state=100, reg_alpha=0, reg_lambda=1, scale_pos_weight=1, subsample=0.5, tree_method='exact', validate_parameters=1, verbosity=None)
- 중요 변수
model.feature_importances_
array([0.038919 , 0.01267713, 0.01225787, 0.01050511, 0.01163761, 0.01353427, 0.00933271, 0.01493725, 0.01142371, 0.01449663, 0.0147713 , 0.04279777, 0.01034376, 0.01216397, 0.03188165, 0.01175237, 0.02284922, 0.01379388, 0.01223848, 0.0172476 , 0.00816719, 0.01348241, 0.01095861, 0.02659101, 0.01436962, 0.01164547, 0.0322113 , 0.01552235, 0.01792656, 0.0112018 , 0.01060445, 0.01817431, 0.05123057, 0.0214917 , 0.04232735, 0.01247919, 0.01064138, 0.00784212, 0.01521082, 0.01216283, 0.01189425, 0.01306549, 0.01088845, 0.00932857, 0.0105593 , 0.0122491 , 0.01156548, 0.01200127, 0.01363443, 0.01050587, 0.01028389, 0.01061128, 0.02285919, 0.01164282, 0.0304599 , 0.02732371, 0.01601311, 0.01338804, 0.02446358, 0.01346002], dtype=float32)
important_feature = pd.DataFrame({'features': X_train.columns, 'values': model.feature_importances_})
pd.options.display.float_format = '{:.6f}'.format
important_feature.sort_values(by='values', ascending=False)
features | values | |
---|---|---|
32 | like | 0.051231 |
11 | attractive_o | 0.042798 |
34 | met | 0.042327 |
0 | has_null | 0.038919 |
26 | funny_partner | 0.032211 |
14 | funny_o | 0.031882 |
54 | race_Latino/HispanicAmerican | 0.030460 |
55 | race_Other | 0.027324 |
23 | attractive_partner | 0.026591 |
58 | race_o_Latino/HispanicAmerican | 0.024464 |
52 | race_Black/AfricanAmerican | 0.022859 |
16 | shared_interests_o | 0.022849 |
33 | guess_prob_liked | 0.021492 |
31 | expected_num_interested_in_me | 0.018174 |
28 | shared_interests_partner | 0.017927 |
19 | intellicence_important | 0.017248 |
56 | race_o_Black/AfricanAmerican | 0.016013 |
27 | ambition_partner | 0.015522 |
38 | same_race_point | 0.015211 |
7 | pref_o_intelligence | 0.014937 |
10 | pref_o_shared_interests | 0.014771 |
9 | pref_o_ambitious | 0.014497 |
24 | sincere_partner | 0.014370 |
17 | attractive_important | 0.013794 |
48 | funny_me | 0.013634 |
5 | pref_o_attractive | 0.013534 |
21 | ambtition_important | 0.013482 |
59 | race_o_Other | 0.013460 |
57 | race_o_European/Caucasian-American | 0.013388 |
41 | intelligence_ptnr | 0.013065 |
1 | age | 0.012677 |
35 | age_gap | 0.012479 |
2 | age_o | 0.012258 |
45 | attractive_me | 0.012249 |
18 | sincere_important | 0.012238 |
13 | intelligence_o | 0.012164 |
39 | attractive_ptnr | 0.012163 |
47 | intelligence_me | 0.012001 |
40 | sincere_ptnr | 0.011894 |
15 | ambitous_o | 0.011752 |
25 | intelligence_partner | 0.011645 |
53 | race_European/Caucasian-American | 0.011643 |
4 | importance_same_religion | 0.011638 |
46 | sincere_me | 0.011565 |
8 | pref_o_funny | 0.011424 |
29 | interests_correlate | 0.011202 |
22 | shared_interests_important | 0.010959 |
42 | funny_ptnr | 0.010888 |
36 | age_gap_abs | 0.010641 |
51 | gender_male | 0.010611 |
30 | expected_happy_with_sd_people | 0.010604 |
44 | shared_ptnr | 0.010559 |
49 | ambition_me | 0.010506 |
3 | importance_same_race | 0.010505 |
12 | sincere_o | 0.010344 |
50 | shared_me | 0.010284 |
6 | pref_o_sincere | 0.009333 |
43 | ambition_ptnr | 0.009329 |
20 | funny_important | 0.008167 |
37 | same_race | 0.007842 |
plt.figure(figsize=(100, 200))
important_feature.sort_values(by='values', ascending=True).plot(kind='bar')
plt.show()
<Figure size 10000x20000 with 0 Axes>
plt.figure(figsize=(20, 10))
sns.barplot(
x='values', y='features', data=important_feature.sort_values(by='values', ascending=False).head()
)
plt.show()
'CS & DS > scikit-learn Machine Learning' 카테고리의 다른 글
scikit-learn Machine Learning K-Means Clustering 사이킷런 머신러닝 K-평균 군집화 (0) | 2022.11.21 |
---|---|
scikit-learn Machine Learning LightGBM 사이킷런 머신러닝 LightGBM (0) | 2022.11.21 |
scikit-learn Machine Learning RandomForest 사이킷런 머신러닝 랜덤포레스트 (0) | 2022.11.21 |
scikit-learn Machine Learning DecisionTree 사이킷런 머신러닝 결정트리 (0) | 2022.11.21 |
scikit-learn Machine Learning Naive Bayes Kor NLP 사이킷런 머신러닝 나이브베이즈 한글 자연어처리 (0) | 2022.11.21 |