일 | 월 | 화 | 수 | 목 | 금 | 토 |
---|---|---|---|---|---|---|
1 | 2 | 3 | 4 | 5 | ||
6 | 7 | 8 | 9 | 10 | 11 | 12 |
13 | 14 | 15 | 16 | 17 | 18 | 19 |
20 | 21 | 22 | 23 | 24 | 25 | 26 |
27 | 28 | 29 | 30 |
- K평균군집화
- 파이썬
- scikit-learn
- sklearn
- Titanic data set
- pandas
- NumPy
- 파이썬 제어문
- Python crawler
- 사이킷런
- 배열
- 판다스
- Machine Learning
- 타이타닉 데이터
- Naive Bayes
- KMeans Clustering
- dataframe
- python control statement
- 순회 크롤러
- 파이썬 객체 지향 프로그래밍
- 머신러닝
- ML
- 파이썬 크롤링
- control statement
- python
- 넘파이
- 나이브베이즈
- 파이썬 크롤러
- Data pre-processing
- 제어문
- Today
- Total
Try to 개발자 EthanJ의 성장 로그
scikit-learn Machine Learning LightGBM 사이킷런 머신러닝 LightGBM 본문
scikit-learn Machine Learning LightGBM 사이킷런 머신러닝 LightGBM
EthanJ 2022. 11. 21. 14:51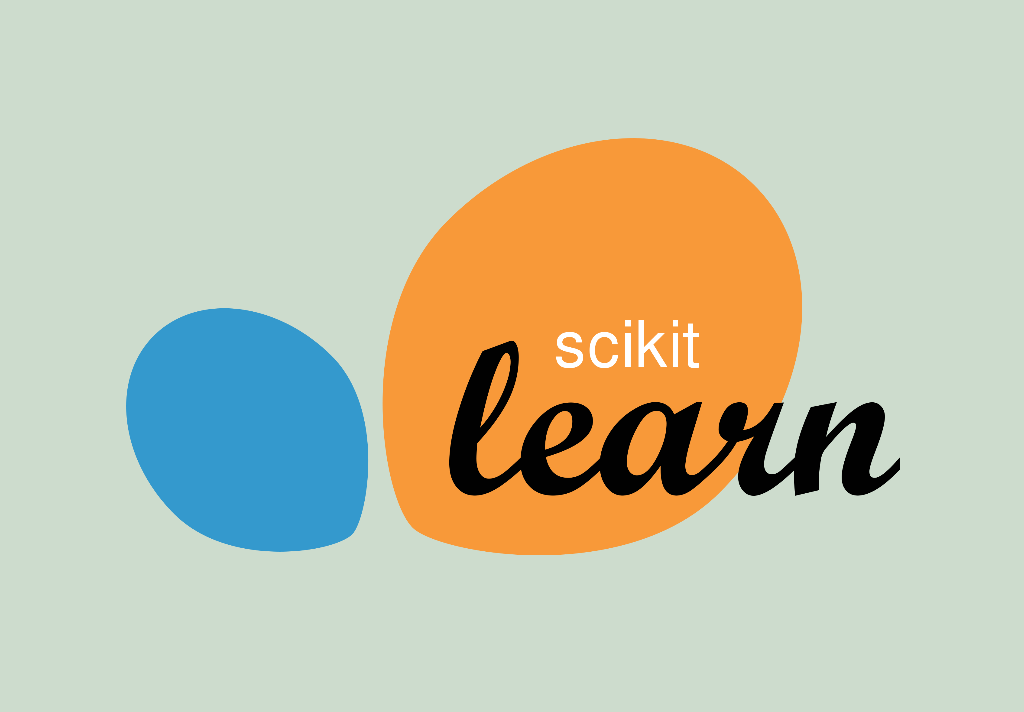
scikit-learn Machine Learning LightGBM
사이킷런 머신러닝 LightGBM
import pandas as pd
import numpy as np
import matplotlib.pyplot as plt
import seaborn as sns
Data Collection¶
# https://www.kaggle.com/datasets/kartik2112/fraud-detection
file_url = 'https://media.githubusercontent.com/media/musthave-ML10/data_source/main/fraud.csv'
df = pd.read_csv(file_url)
df.head()
trans_date_trans_time | cc_num | merchant | category | amt | first | last | gender | street | city | ... | lat | long | city_pop | job | dob | trans_num | unix_time | merch_lat | merch_long | is_fraud | |
---|---|---|---|---|---|---|---|---|---|---|---|---|---|---|---|---|---|---|---|---|---|
0 | 2019-01-01 00:00:18 | 2703186189652095 | fraud_Rippin, Kub and Mann | misc_net | 4.97 | Jennifer | Banks | F | 561 Perry Cove | Moravian Falls | ... | 36.0788 | -81.1781 | 3495 | Psychologist, counselling | 1988-03-09 | 0b242abb623afc578575680df30655b9 | 1325376018 | 36.011293 | -82.048315 | 0 |
1 | 2019-01-01 00:00:44 | 630423337322 | fraud_Heller, Gutmann and Zieme | grocery_pos | 107.23 | Stephanie | Gill | F | 43039 Riley Greens Suite 393 | Orient | ... | 48.8878 | -118.2105 | 149 | Special educational needs teacher | 1978-06-21 | 1f76529f8574734946361c461b024d99 | 1325376044 | 49.159047 | -118.186462 | 0 |
2 | 2019-01-01 00:00:51 | 38859492057661 | fraud_Lind-Buckridge | entertainment | 220.11 | Edward | Sanchez | M | 594 White Dale Suite 530 | Malad City | ... | 42.1808 | -112.2620 | 4154 | Nature conservation officer | 1962-01-19 | a1a22d70485983eac12b5b88dad1cf95 | 1325376051 | 43.150704 | -112.154481 | 0 |
3 | 2019-01-01 00:01:16 | 3534093764340240 | fraud_Kutch, Hermiston and Farrell | gas_transport | 45.00 | Jeremy | White | M | 9443 Cynthia Court Apt. 038 | Boulder | ... | 46.2306 | -112.1138 | 1939 | Patent attorney | 1967-01-12 | 6b849c168bdad6f867558c3793159a81 | 1325376076 | 47.034331 | -112.561071 | 0 |
4 | 2019-01-01 00:03:06 | 375534208663984 | fraud_Keeling-Crist | misc_pos | 41.96 | Tyler | Garcia | M | 408 Bradley Rest | Doe Hill | ... | 38.4207 | -79.4629 | 99 | Dance movement psychotherapist | 1986-03-28 | a41d7549acf90789359a9aa5346dcb46 | 1325376186 | 38.674999 | -78.632459 | 0 |
5 rows × 22 columns
df.columns
Index(['trans_date_trans_time', 'cc_num', 'merchant', 'category', 'amt', 'first', 'last', 'gender', 'street', 'city', 'state', 'zip', 'lat', 'long', 'city_pop', 'job', 'dob', 'trans_num', 'unix_time', 'merch_lat', 'merch_long', 'is_fraud'], dtype='object')
trans_date_trans_time
: 거래 시간cc_num
: 카드 번호. 고윳값이기 때문에 여기서는 id처럼 활용할 수 있음merchant
: 거래 상점category
: 거래 상점의 범주(애완용품, 여행, 엔터테인먼트 등)amt
: 거래금액 (amount)first
,last
: 이름gender
: 성별street
,state
,zip
: 고객 거주지 정보lat
,long
: 고객주소에 대한 위도 및 경도city_pop
: 고객의 zipcode 속하는 인구 수job
: 직업dob
: 생년월일trans_num
: 거래번호unix_time
: 거래시간 (유닉스 타임스탬프)merch_lat
,merch_long
: 상점의 위경도is_fraud
: 사기거래 여부 (이상거래 여부): target
df.info()
<class 'pandas.core.frame.DataFrame'> RangeIndex: 1852394 entries, 0 to 1852393 Data columns (total 22 columns): # Column Dtype --- ------ ----- 0 trans_date_trans_time object 1 cc_num int64 2 merchant object 3 category object 4 amt float64 5 first object 6 last object 7 gender object 8 street object 9 city object 10 state object 11 zip int64 12 lat float64 13 long float64 14 city_pop int64 15 job object 16 dob object 17 trans_num object 18 unix_time int64 19 merch_lat float64 20 merch_long float64 21 is_fraud int64 dtypes: float64(5), int64(5), object(12) memory usage: 310.9+ MB
df.info(show_counts=True)
<class 'pandas.core.frame.DataFrame'> RangeIndex: 1852394 entries, 0 to 1852393 Data columns (total 22 columns): # Column Non-Null Count Dtype --- ------ -------------- ----- 0 trans_date_trans_time 1852394 non-null object 1 cc_num 1852394 non-null int64 2 merchant 1852394 non-null object 3 category 1852394 non-null object 4 amt 1852394 non-null float64 5 first 1852394 non-null object 6 last 1852394 non-null object 7 gender 1852394 non-null object 8 street 1852394 non-null object 9 city 1852394 non-null object 10 state 1852394 non-null object 11 zip 1852394 non-null int64 12 lat 1852394 non-null float64 13 long 1852394 non-null float64 14 city_pop 1852394 non-null int64 15 job 1852394 non-null object 16 dob 1852394 non-null object 17 trans_num 1852394 non-null object 18 unix_time 1852394 non-null int64 19 merch_lat 1852394 non-null float64 20 merch_long 1852394 non-null float64 21 is_fraud 1852394 non-null int64 dtypes: float64(5), int64(5), object(12) memory usage: 310.9+ MB
pd.options.display.float_format = '{:.2f}'.format
df.describe()
cc_num | amt | zip | lat | long | city_pop | unix_time | merch_lat | merch_long | is_fraud | |
---|---|---|---|---|---|---|---|---|---|---|
count | 1852394.00 | 1852394.00 | 1852394.00 | 1852394.00 | 1852394.00 | 1852394.00 | 1852394.00 | 1852394.00 | 1852394.00 | 1852394.00 |
mean | 417386038394006464.00 | 70.06 | 48813.26 | 38.54 | -90.23 | 88643.67 | 1358674218.83 | 38.54 | -90.23 | 0.01 |
std | 1309115265318020352.00 | 159.25 | 26881.85 | 5.07 | 13.75 | 301487.62 | 18195081.39 | 5.11 | 13.76 | 0.07 |
min | 60416207185.00 | 1.00 | 1257.00 | 20.03 | -165.67 | 23.00 | 1325376018.00 | 19.03 | -166.67 | 0.00 |
25% | 180042946491150.00 | 9.64 | 26237.00 | 34.67 | -96.80 | 741.00 | 1343016823.75 | 34.74 | -96.90 | 0.00 |
50% | 3521417320836166.00 | 47.45 | 48174.00 | 39.35 | -87.48 | 2443.00 | 1357089331.00 | 39.37 | -87.44 | 0.00 |
75% | 4642255475285942.00 | 83.10 | 72042.00 | 41.94 | -80.16 | 20328.00 | 1374581485.25 | 41.96 | -80.25 | 0.00 |
max | 4992346398065154048.00 | 28948.90 | 99921.00 | 66.69 | -67.95 | 2906700.00 | 1388534374.00 | 67.51 | -66.95 | 1.00 |
Data pre-processing¶
의미 없는 Data 제거¶
df = df.drop(columns=['first', 'last', 'street', 'city', 'state', 'zip',
'trans_num', 'unix_time', 'job', 'merchant'])
df.columns
Index(['trans_date_trans_time', 'cc_num', 'category', 'amt', 'gender', 'lat', 'long', 'city_pop', 'dob', 'merch_lat', 'merch_long', 'is_fraud'], dtype='object')
df['trans_date_trans_time'] = pd.to_datetime(df['trans_date_trans_time'])
df.info()
<class 'pandas.core.frame.DataFrame'> RangeIndex: 1852394 entries, 0 to 1852393 Data columns (total 12 columns): # Column Dtype --- ------ ----- 0 trans_date_trans_time datetime64[ns] 1 cc_num int64 2 category object 3 amt float64 4 gender object 5 lat float64 6 long float64 7 city_pop int64 8 dob object 9 merch_lat float64 10 merch_long float64 11 is_fraud int64 dtypes: datetime64[ns](1), float64(5), int64(3), object(3) memory usage: 169.6+ MB
feature engineering¶
- 고객의 원래 거래 패턴에서 벗어나는 거래 탐지
결제 금액¶
정규분포 Z점수: $\frac{x-\bar{x}}{\sigma}$
df.groupby().agg([list of value])
: 계산된 통계값을 묶어서 표현mean
: 평균,std
: 표준편차,amt
: 결제금액
# 각 카드번호별 '결제금액'의 평균, 표준편차
amt_info = df.groupby('cc_num').agg(['mean', 'std'])['amt'].reset_index()
amt_info.head()
C:\Users\EthanJ\AppData\Local\Temp\ipykernel_14708\3458248078.py:2: FutureWarning: ['trans_date_trans_time', 'category', 'gender', 'dob'] did not aggregate successfully. If any error is raised this will raise in a future version of pandas. Drop these columns/ops to avoid this warning. amt_info = df.groupby('cc_num').agg(['mean', 'std'])['amt'].reset_index()
cc_num | mean | std | |
---|---|---|---|
0 | 60416207185 | 59.26 | 142.87 |
1 | 60422928733 | 65.48 | 92.04 |
2 | 60423098130 | 96.38 | 1000.69 |
3 | 60427851591 | 107.49 | 131.01 |
4 | 60487002085 | 64.10 | 153.21 |
df = df.merge(amt_info, on='cc_num', how='left')
df.columns
Index(['trans_date_trans_time', 'cc_num', 'category', 'amt', 'gender', 'lat', 'long', 'city_pop', 'dob', 'merch_lat', 'merch_long', 'is_fraud', 'mean', 'std'], dtype='object')
df[['cc_num', 'amt', 'mean', 'std']].head()
cc_num | amt | mean | std | |
---|---|---|---|---|
0 | 2703186189652095 | 4.97 | 89.41 | 127.53 |
1 | 630423337322 | 107.23 | 56.08 | 159.20 |
2 | 38859492057661 | 220.11 | 69.92 | 116.69 |
3 | 3534093764340240 | 45.00 | 80.09 | 280.08 |
4 | 375534208663984 | 41.96 | 95.34 | 94.32 |
df['amt_z_score'] = (df['amt'] - df['mean']) / df['std']
df['amt_z_score'].head()
0 -0.66 1 0.32 2 1.29 3 -0.13 4 -0.57 Name: amt_z_score, dtype: float64
df = df.drop(columns=['mean', 'std'])
df.columns
Index(['trans_date_trans_time', 'cc_num', 'category', 'amt', 'gender', 'lat', 'long', 'city_pop', 'dob', 'merch_lat', 'merch_long', 'is_fraud', 'amt_z_score'], dtype='object')
category
별 amt
(결제금액)¶
category_info = df.groupby(['cc_num', 'category']).agg(['mean', 'std'])['amt'].reset_index()
category_info.head()
C:\Users\EthanJ\AppData\Local\Temp\ipykernel_14708\2233020675.py:1: FutureWarning: ['trans_date_trans_time', 'gender', 'dob'] did not aggregate successfully. If any error is raised this will raise in a future version of pandas. Drop these columns/ops to avoid this warning. category_info = df.groupby(['cc_num', 'category']).agg(['mean', 'std'])['amt'].reset_index()
cc_num | category | mean | std | |
---|---|---|---|---|
0 | 60416207185 | entertainment | 51.84 | 65.49 |
1 | 60416207185 | food_dining | 26.74 | 46.38 |
2 | 60416207185 | gas_transport | 59.78 | 15.76 |
3 | 60416207185 | grocery_net | 52.15 | 17.69 |
4 | 60416207185 | grocery_pos | 101.56 | 21.89 |
df = df.merge(category_info, on=['cc_num', 'category'], how='left')
df.columns
Index(['trans_date_trans_time', 'cc_num', 'category', 'amt', 'gender', 'lat', 'long', 'city_pop', 'dob', 'merch_lat', 'merch_long', 'is_fraud', 'amt_z_score', 'mean', 'std'], dtype='object')
df[['cc_num', 'category', 'amt', 'mean', 'std']].head()
cc_num | category | amt | mean | std | |
---|---|---|---|---|---|
0 | 2703186189652095 | misc_net | 4.97 | 84.86 | 116.07 |
1 | 630423337322 | grocery_pos | 107.23 | 99.64 | 23.90 |
2 | 38859492057661 | entertainment | 220.11 | 46.65 | 60.39 |
3 | 3534093764340240 | gas_transport | 45.00 | 61.54 | 15.75 |
4 | 375534208663984 | misc_pos | 41.96 | 35.48 | 4.93 |
df['cate_z_score'] = (df['amt'] - df['mean']) / df['std']
df['cate_z_score'].head()
0 -0.69 1 0.32 2 2.87 3 -1.05 4 1.31 Name: cate_z_score, dtype: float64
df = df.drop(columns=['mean', 'std'])
df.columns
Index(['trans_date_trans_time', 'cc_num', 'category', 'amt', 'gender', 'lat', 'long', 'city_pop', 'dob', 'merch_lat', 'merch_long', 'is_fraud', 'amt_z_score', 'cate_z_score'], dtype='object')
distance
¶
import geopy.distance
두 지점간의 거리 (위도, 경도)
geopy.distance.distance((lat1, long1), (lat2, long2))
coordinate
좌표, 위치: (위도, 경도)상점의 위치: merchant_coordinate >
merch_coor
고객의 위치: customer_coordinate >
custo_coor
df['merch_coor'] = pd.Series(zip(df['merch_lat'], df['merch_long']))
df['custo_coor'] = pd.Series(zip(df['lat'], df['long']))
df['distance'] = df.apply(lambda x: geopy.distance.distance(x['merch_coor'], x['custo_coor']).km, axis=1)
df['distance'].head()
0 78.77 1 30.22 2 108.10 3 95.69 4 77.70 Name: distance, dtype: float64
distance().km
: float data type
distance_info = df.groupby('cc_num').agg(['mean', 'std'])['distance'].reset_index()
distance_info.head()
C:\Users\EthanJ\AppData\Local\Temp\ipykernel_14708\2201206611.py:1: FutureWarning: ['trans_date_trans_time', 'category', 'gender', 'dob', 'merch_coor', 'custo_coor'] did not aggregate successfully. If any error is raised this will raise in a future version of pandas. Drop these columns/ops to avoid this warning. distance_info = df.groupby('cc_num').agg(['mean', 'std'])['distance'].reset_index()
cc_num | mean | std | |
---|---|---|---|
0 | 60416207185 | 73.53 | 28.70 |
1 | 60422928733 | 78.99 | 29.30 |
2 | 60423098130 | 77.83 | 28.19 |
3 | 60427851591 | 75.71 | 28.98 |
4 | 60487002085 | 79.44 | 28.77 |
df = df.merge(distance_info, on='cc_num', how='left')
df['distance_z_score'] = (df['distance'] - df['mean']) / df['std']
df['distance_z_score'].head()
0 0.03 1 -1.48 2 1.16 3 0.82 4 0.06 Name: distance_z_score, dtype: float64
df = df.drop(['mean', 'std'], axis=1)
df.head()
trans_date_trans_time | cc_num | category | amt | gender | lat | long | city_pop | dob | merch_lat | merch_long | is_fraud | amt_z_score | cate_z_score | merch_coor | custo_coor | distance | distance_z_score | |
---|---|---|---|---|---|---|---|---|---|---|---|---|---|---|---|---|---|---|
0 | 2019-01-01 00:00:18 | 2703186189652095 | misc_net | 4.97 | F | 36.08 | -81.18 | 3495 | 1988-03-09 | 36.01 | -82.05 | 0 | -0.66 | -0.69 | (36.011293, -82.048315) | (36.0788, -81.1781) | 78.77 | 0.03 |
1 | 2019-01-01 00:00:44 | 630423337322 | grocery_pos | 107.23 | F | 48.89 | -118.21 | 149 | 1978-06-21 | 49.16 | -118.19 | 0 | 0.32 | 0.32 | (49.159047, -118.186462) | (48.8878, -118.2105) | 30.22 | -1.48 |
2 | 2019-01-01 00:00:51 | 38859492057661 | entertainment | 220.11 | M | 42.18 | -112.26 | 4154 | 1962-01-19 | 43.15 | -112.15 | 0 | 1.29 | 2.87 | (43.150704, -112.154481) | (42.1808, -112.262) | 108.10 | 1.16 |
3 | 2019-01-01 00:01:16 | 3534093764340240 | gas_transport | 45.00 | M | 46.23 | -112.11 | 1939 | 1967-01-12 | 47.03 | -112.56 | 0 | -0.13 | -1.05 | (47.034331, -112.561071) | (46.2306, -112.1138) | 95.69 | 0.82 |
4 | 2019-01-01 00:03:06 | 375534208663984 | misc_pos | 41.96 | M | 38.42 | -79.46 | 99 | 1986-03-28 | 38.67 | -78.63 | 0 | -0.57 | 1.31 | (38.674999, -78.632459) | (38.4207, -79.4629) | 77.70 | 0.06 |
df = df.drop(columns=['cc_num', 'lat', 'long', 'merch_lat', 'merch_long', 'merch_coor', 'custo_coor'])
df.columns
Index(['trans_date_trans_time', 'category', 'amt', 'gender', 'city_pop', 'dob', 'is_fraud', 'amt_z_score', 'cate_z_score', 'distance', 'distance_z_score'], dtype='object')
df.isna().sum()
trans_date_trans_time 0 category 0 amt 0 gender 0 city_pop 0 dob 0 is_fraud 0 amt_z_score 0 cate_z_score 219 distance 0 distance_z_score 0 dtype: int64
dob
> age
¶
df['dob'].head()
0 1988-03-09 1 1978-06-21 2 1962-01-19 3 1967-01-12 4 1986-03-28 Name: dob, dtype: object
df['dob'] = pd.to_datetime(df['dob'])
df['dob']
0 1988-03-09 1 1978-06-21 2 1962-01-19 3 1967-01-12 4 1986-03-28 ... 1852389 1966-02-13 1852390 1999-12-27 1852391 1981-11-29 1852392 1965-12-15 1852393 1993-05-10 Name: dob, Length: 1852394, dtype: datetime64[ns]
# 만나이 계산
df['age'] = 2021 - df['dob'].dt.year
df['age']
0 33 1 43 2 59 3 54 4 35 .. 1852389 55 1852390 22 1852391 40 1852392 56 1852393 28 Name: age, Length: 1852394, dtype: int64
df = df.drop(columns=['dob'])
df.info()
<class 'pandas.core.frame.DataFrame'> Int64Index: 1852394 entries, 0 to 1852393 Data columns (total 11 columns): # Column Dtype --- ------ ----- 0 trans_date_trans_time datetime64[ns] 1 category object 2 amt float64 3 gender object 4 city_pop int64 5 is_fraud int64 6 amt_z_score float64 7 cate_z_score float64 8 distance float64 9 distance_z_score float64 10 age int64 dtypes: datetime64[ns](1), float64(5), int64(3), object(2) memory usage: 169.6+ MB
get_dummies()
¶
df = pd.get_dummies(df, columns=['category', 'gender'], drop_first=True)
df.tail()
trans_date_trans_time | amt | city_pop | is_fraud | amt_z_score | cate_z_score | distance | distance_z_score | age | category_food_dining | ... | category_health_fitness | category_home | category_kids_pets | category_misc_net | category_misc_pos | category_personal_care | category_shopping_net | category_shopping_pos | category_travel | gender_M | |
---|---|---|---|---|---|---|---|---|---|---|---|---|---|---|---|---|---|---|---|---|---|
1852389 | 2020-12-31 23:59:07 | 43.77 | 519 | 0 | -0.17 | -0.05 | 77.03 | 0.05 | 55 | 0 | ... | 1 | 0 | 0 | 0 | 0 | 0 | 0 | 0 | 0 | 1 |
1852390 | 2020-12-31 23:59:09 | 111.84 | 28739 | 0 | 0.36 | 1.16 | 100.02 | 0.69 | 22 | 0 | ... | 0 | 0 | 1 | 0 | 0 | 0 | 0 | 0 | 0 | 1 |
1852391 | 2020-12-31 23:59:15 | 86.88 | 3684 | 0 | -0.02 | 0.44 | 80.89 | 0.29 | 40 | 0 | ... | 0 | 0 | 1 | 0 | 0 | 0 | 0 | 0 | 0 | 0 |
1852392 | 2020-12-31 23:59:24 | 7.99 | 129 | 0 | -0.59 | -0.01 | 53.06 | -0.73 | 56 | 0 | ... | 0 | 0 | 0 | 0 | 0 | 0 | 0 | 0 | 1 | 1 |
1852393 | 2020-12-31 23:59:34 | 38.13 | 116001 | 0 | -0.15 | -0.38 | 72.38 | -0.16 | 28 | 0 | ... | 0 | 0 | 0 | 0 | 0 | 0 | 0 | 0 | 0 | 1 |
5 rows × 23 columns
df.info()
<class 'pandas.core.frame.DataFrame'> Int64Index: 1852394 entries, 0 to 1852393 Data columns (total 23 columns): # Column Dtype --- ------ ----- 0 trans_date_trans_time datetime64[ns] 1 amt float64 2 city_pop int64 3 is_fraud int64 4 amt_z_score float64 5 cate_z_score float64 6 distance float64 7 distance_z_score float64 8 age int64 9 category_food_dining uint8 10 category_gas_transport uint8 11 category_grocery_net uint8 12 category_grocery_pos uint8 13 category_health_fitness uint8 14 category_home uint8 15 category_kids_pets uint8 16 category_misc_net uint8 17 category_misc_pos uint8 18 category_personal_care uint8 19 category_shopping_net uint8 20 category_shopping_pos uint8 21 category_travel uint8 22 gender_M uint8 dtypes: datetime64[ns](1), float64(5), int64(3), uint8(14) memory usage: 166.1 MB
set_index()
¶
df = df.set_index('trans_date_trans_time')
df.index
DatetimeIndex(['2019-01-01 00:00:18', '2019-01-01 00:00:44', '2019-01-01 00:00:51', '2019-01-01 00:01:16', '2019-01-01 00:03:06', '2019-01-01 00:04:08', '2019-01-01 00:04:42', '2019-01-01 00:05:08', '2019-01-01 00:05:18', '2019-01-01 00:06:01', ... '2020-12-31 23:57:18', '2020-12-31 23:57:50', '2020-12-31 23:57:56', '2020-12-31 23:58:04', '2020-12-31 23:58:34', '2020-12-31 23:59:07', '2020-12-31 23:59:09', '2020-12-31 23:59:15', '2020-12-31 23:59:24', '2020-12-31 23:59:34'], dtype='datetime64[ns]', name='trans_date_trans_time', length=1852394, freq=None)
Training Model¶
from sklearn.model_selection import train_test_split
X = df.drop(columns=['is_fraud'])
y = df['is_fraud']
X_train, X_test, y_train, y_test = train_test_split(
X, y, test_size = 0.2, random_state = 814
)
import lightgbm as lgb
model = lgb.LGBMClassifier(random_state=np.random.randint(1000))
model.fit(X_train, y_train)
pred = model.predict(X_test)
Evaluating Model¶
from sklearn.metrics import accuracy_score
accuracy_score(y_test, pred)
0.9966016967223513
- Data의 편향성
1 - df['is_fraud'].mean()
0.9947899852839083
Visualizing Data¶
from sklearn.metrics import confusion_matrix, classification_report, roc_auc_score
cf_matrix = confusion_matrix(y_test, pred)
print(cf_matrix)
[[368030 558] [ 701 1190]]
group_name = ['TN', 'FP', 'FN', 'TP']
group_counts = ["{0:0.0f}".format(value) for value in cf_matrix.flatten()]
group_percent = ["{0:.2%}".format(value) for value in (cf_matrix.flatten() / np.sum(cf_matrix))]
labels = [f"{name}\n{counts}\n{percent}" for name, counts, percent in
zip(group_name, group_counts, group_percent)]
labels = np.asarray(labels).reshape(2, 2)
sns.heatmap(cf_matrix, annot=labels, fmt='', cmap='coolwarm')
plt.ylabel('Actual')
plt.xlabel('Predicted')
plt.show()
precision
정밀도: 1종 오류recall
재현도: 2종 오류f1-score
: 전체
print(classification_report(y_test, pred))
precision recall f1-score support 0 1.00 1.00 1.00 368588 1 0.68 0.63 0.65 1891 accuracy 1.00 370479 macro avg 0.84 0.81 0.83 370479 weighted avg 1.00 1.00 1.00 370479
model.predict_proba()
¶
- 분류되기 전에 얼만큼의 확률을 보였는지 나타냄
proba = model.predict_proba(X_test)
proba
array([[9.99069879e-01, 9.30120853e-04], [9.99957475e-01, 4.25253222e-05], [9.99929953e-01, 7.00468547e-05], ..., [9.97326245e-01, 2.67375455e-03], [9.99684243e-01, 3.15757316e-04], [9.99924891e-01, 7.51086766e-05]])
각각의 행: 입력받은 test data별
1열: 0에 대한 예측값(target이 아닐 예측)
2열: 1에 대한 예측값(target일 예측)
- 모든 행의 2열: 1에 대한 예측값: target(이상거래)
proba[:, 1]
array([9.30120853e-04, 4.25253222e-05, 7.00468547e-05, ..., 2.67375455e-03, 3.15757316e-04, 7.51086766e-05])
proba = proba[:, 1]
default:
0.5
→ 50% 초과면 1로 인정0.2
: 20%만 초과해도 1로 인정0.8
: 80%보다 초과해야 1로 인정
True
,False
→1
,0
: int
proba_case1 = (proba > 0.2).astype('int')
proba_case2 = (proba > 0.8).astype('int')
def confusion_matrix_view(y_test, pred):
cf_matrix = confusion_matrix(y_test, pred)
print(cf_matrix)
group_names = ['TN','FP','FN','TP']
group_counts = ["{0:0.0f}".format(value) for value in
cf_matrix.flatten()]
group_percentages = ["{0:.2%}".format(value) for value in
cf_matrix.flatten()/np.sum(cf_matrix)]
labels = [f"{v1}\n{v2}\n{v3}" for v1, v2, v3 in
zip(group_names,group_counts,group_percentages)]
labels = np.asarray(labels).reshape(2,2)
sns.heatmap(cf_matrix, annot=labels, fmt='', cmap='coolwarm')
plt.ylabel('Actual')
plt.xlabel('Predicted')
plt.show()
print(classification_report(y_test, pred))
confusion_matrix_view(y_test, pred)
[[368030 558] [ 701 1190]]
precision recall f1-score support 0 1.00 1.00 1.00 368588 1 0.68 0.63 0.65 1891 accuracy 1.00 370479 macro avg 0.84 0.81 0.83 370479 weighted avg 1.00 1.00 1.00 370479
- case1)
0.2
초과면1
: 1종오류 증가, 2종오류 감소
confusion_matrix_view(y_test, proba_case1)
[[367529 1059] [ 552 1339]]
precision recall f1-score support 0 1.00 1.00 1.00 368588 1 0.56 0.71 0.62 1891 accuracy 1.00 370479 macro avg 0.78 0.85 0.81 370479 weighted avg 1.00 1.00 1.00 370479
- case2)
0.8
초과면1
: 1종오류 감소, 2종오류 증가
confusion_matrix_view(y_test, proba_case2)
[[368283 305] [ 933 958]]
precision recall f1-score support 0 1.00 1.00 1.00 368588 1 0.76 0.51 0.61 1891 accuracy 1.00 370479 macro avg 0.88 0.75 0.80 370479 weighted avg 1.00 1.00 1.00 370479
ROC 곡선 & AUC¶
binary classifier model을 평가하는 방법, 기준점에 영향을 받지 않기 때문에 여러 모델을 비교할 때 사용
AUC: ROC 곡선의 아래 면적 →
0.5
~1
, 값이 클수록 좋은 model
기준점을 바꿨을 때,
TPR
,FPR
이 바뀌는 모양을 나타낸 그래프최악의 경우 (학습이 전혀 안 될 경우): 빨간색 점선
AUC (Area Under the ROC Curve)¶
ROC 곡선의 아래쪽에 해당하는 면적
0.5
~1
사이의 값을 가지며 값이 클수록 해당 model이 더 좋은 classifier라는 의미
RandomGridSearch¶
GridSearch: 모든 조합에 대해 modeling
- RandomGridSearch: 더 넓은 영역의 hyperparamter값을 더 짧은 시간에 다양하게 활용 → 일부만 추출해서 사용
from sklearn.model_selection import RandomizedSearchCV
parameters = {
'n_estimators': [100, 500, 1000],
'learning_rate': [0.01, 0.05, 0.1, 0.25],
'lambda_l1': [0, 10, 20, 30, 50],
'lambda_l2': [0, 10, 20, 30, 50],
'max_depth': [5, 10, 15, 20],
'subsample': [0.5, 0.75, 1]
}
L1 정규화, L2 정규화¶
라쏘 회귀(lasso regression): L1 정규화(
lambda_l1
)릿지 회귀(ridge regression): L2 정규화(
lambda_l2
)
매개변수에 패널티를 가해서 영향력(계수)를 감소시키는 방법. 오버피팅 방지 목적.
new_model = lgb.LGBMClassifier(random_state=np.random.randint(1000))
rsCV = RandomizedSearchCV(new_model, param_distributions=parameters, n_iter=30,
scoring='roc_auc', random_state=np.random.randint(1000), n_jobs=6)
import time
start = time.time()
rsCV.fit(X_train, y_train)
print(time.time() - start)
[LightGBM] [Warning] lambda_l1 is set=0, reg_alpha=0.0 will be ignored. Current value: lambda_l1=0 [LightGBM] [Warning] lambda_l2 is set=50, reg_lambda=0.0 will be ignored. Current value: lambda_l2=50 1051.4817752838135
rsCV.best_params_
{'subsample': 0.5, 'n_estimators': 500, 'max_depth': 20, 'learning_rate': 0.25, 'lambda_l2': 50, 'lambda_l1': 0}
rsCV_proba = rsCV.predict_proba(X_test)
roc_auc_score(y_test, rsCV_proba[:, 1])
0.9964346064160456
rsCV_proba_int = (rsCV_proba[:, 1] > 0.2).astype('int')
confusion_matrix_view(y_test, rsCV_proba_int)
[[367768 820] [ 358 1533]]
precision recall f1-score support 0 1.00 1.00 1.00 368588 1 0.65 0.81 0.72 1891 accuracy 1.00 370479 macro avg 0.83 0.90 0.86 370479 weighted avg 1.00 1.00 1.00 370479
confusion_matrix_view(y_test, proba_case1)
[[367529 1059] [ 552 1339]]
precision recall f1-score support 0 1.00 1.00 1.00 368588 1 0.56 0.71 0.62 1891 accuracy 1.00 370479 macro avg 0.78 0.85 0.81 370479 weighted avg 1.00 1.00 1.00 370479
confusion_matrix_view(y_test, proba_case2)
[[368283 305] [ 933 958]]
precision recall f1-score support 0 1.00 1.00 1.00 368588 1 0.76 0.51 0.61 1891 accuracy 1.00 370479 macro avg 0.88 0.75 0.80 370479 weighted avg 1.00 1.00 1.00 370479
train()
method¶
model.fit(X_train, y_train)
→ output: 학습model.train()
← input: fit
train | fit | |
---|---|---|
test set | modeling - test set | test X |
Data Set | DataFrame → 별도 format 변환 | DataFrame, Series |
Hyperparameter | default X | default O |
scikit-learn 연동 | X | O |
fit
: 학습 → train set → model → test settrain
: 학습 → train set과 별개의 validation set → model
def get_X_y(df):
X = df.drop(columns=['is_fraud'])
y = df['is_fraud']
return (X, y)
X, y = get_X_y(df)
X_train, X_test, y_train, y_test = train_test_split(
X, y, test_size=0.2, random_state=814
)
X_train, X_val, y_train, y_val = train_test_split(
X_train, y_train, test_size=0.2, random_state=814
)
data_train = lgb.Dataset(X_train, label=y_train)
data_val = lgb.Dataset(X_val, label=y_val)
param_set = rsCV.best_params_
param_set
{'subsample': 0.5, 'n_estimators': 500, 'max_depth': 20, 'learning_rate': 0.25, 'lambda_l2': 50, 'lambda_l1': 0, 'metrics': 'auc'}
model_train = lgb.train(param_set, data_train, valid_sets=[data_val],
# early_stopping_rounds: 학습 시간 제한
# verbose_eval: 중간결과를 특정 간격으로 출력
early_stopping_rounds=100, verbose_eval=100)
C:\Users\EthanJ\anaconda3\lib\site-packages\lightgbm\engine.py:177: UserWarning: Found `n_estimators` in params. Will use it instead of argument _log_warning(f"Found `{alias}` in params. Will use it instead of argument") C:\Users\EthanJ\anaconda3\lib\site-packages\lightgbm\engine.py:181: UserWarning: 'early_stopping_rounds' argument is deprecated and will be removed in a future release of LightGBM. Pass 'early_stopping()' callback via 'callbacks' argument instead. _log_warning("'early_stopping_rounds' argument is deprecated and will be removed in a future release of LightGBM. " C:\Users\EthanJ\anaconda3\lib\site-packages\lightgbm\engine.py:239: UserWarning: 'verbose_eval' argument is deprecated and will be removed in a future release of LightGBM. Pass 'log_evaluation()' callback via 'callbacks' argument instead. _log_warning("'verbose_eval' argument is deprecated and will be removed in a future release of LightGBM. "
[LightGBM] [Warning] Accuracy may be bad since you didn't explicitly set num_leaves OR 2^max_depth > num_leaves. (num_leaves=31). [LightGBM] [Warning] Accuracy may be bad since you didn't explicitly set num_leaves OR 2^max_depth > num_leaves. (num_leaves=31). [LightGBM] [Warning] Auto-choosing row-wise multi-threading, the overhead of testing was 0.004016 seconds. You can set `force_row_wise=true` to remove the overhead. And if memory is not enough, you can set `force_col_wise=true`. [LightGBM] [Info] Total Bins 1632 [LightGBM] [Info] Number of data points in the train set: 1185532, number of used features: 21 [LightGBM] [Warning] Accuracy may be bad since you didn't explicitly set num_leaves OR 2^max_depth > num_leaves. (num_leaves=31). [LightGBM] [Info] Start training from score 0.005262 Training until validation scores don't improve for 100 rounds [100] valid_0's auc: 0.993644 Early stopping, best iteration is: [97] valid_0's auc: 0.994762
pred_train = model_train.predict(X_test)
roc_auc_score(y_test, pred_train)
0.9929069517179909
feature_imptnt = pd.DataFrame({'features': X_train.columns, 'values': model.feature_importances_})
plt.figure(figsize=(20, 10))
sns.barplot(x='values', y='features',
data=feature_imptnt.sort_values(by='values', ascending=False).head(10))
plt.show()
'CS & DS > scikit-learn Machine Learning' 카테고리의 다른 글
scikit-learn Machine Learning K-Means Clustering 사이킷런 머신러닝 K-평균 군집화 (0) | 2022.11.21 |
---|---|
scikit-learn Machine Learning XGBoost 사이킷런 머신러닝 XGBoost (0) | 2022.11.21 |
scikit-learn Machine Learning RandomForest 사이킷런 머신러닝 랜덤포레스트 (0) | 2022.11.21 |
scikit-learn Machine Learning DecisionTree 사이킷런 머신러닝 결정트리 (0) | 2022.11.21 |
scikit-learn Machine Learning Naive Bayes Kor NLP 사이킷런 머신러닝 나이브베이즈 한글 자연어처리 (0) | 2022.11.21 |